-
Notifications
You must be signed in to change notification settings - Fork 650
New issue
Have a question about this project? Sign up for a free GitHub account to open an issue and contact its maintainers and the community.
By clicking “Sign up for GitHub”, you agree to our terms of service and privacy statement. We’ll occasionally send you account related emails.
Already on GitHub? Sign in to your account
Using jax scan for PPO + atari + envpool XLA #328
Conversation
The latest updates on your projects. Learn more about Vercel for Git ↗︎
|
Thanks for preparing this PR @51616. Out of curiosity, what's the speed difference when running with the following?
|
Here's the training time comparison. I don't think we can compare the code speed by looking purely at this graph because the speed also depends on how fast the agent learns. Since environment reset is relatively expensive, the faster the agent gets better, the fewer resets are called. The rate at which the agent learns depends on exploration that we don't have full control over. Explicitly setting the random seed still cannot precisely reproduce runs. Anyway, I think we shouldn't expect any speed difference between the two versions (jax-ml/jax#402 (comment)). The benefits of this change is mostly the reduced compilation time. The compilation time for the default If you think that jax's idiomatic is not so pythonic and hard to read, we can keep both versions. I think there is value in providing example in jax's idiomatic tools. |
@51616 thanks for the detailed explanation. I really like this prototype and think it's probably worth having both versions as references. On a high level, there are some remaining todos (in chronological orders):
from cleanrl.ppo_atari_envpool_xla_jax_scan import compute_gae
from cleanrl.ppo_atari_envpool_xla_jax import compute_gae
# fakes same data and assert the output from the compute gae functions are the same. After these three steps feel free to ping me to review again, and the last step would be to do the following:
|
@vwxyzjn I did clean up some of the code. Please let me know if there's any specific place I should fix. I have a few questions regarding the tests/benchmarks:
|
Ah my bad for not thinking this through. In that case, maybe don't import the
Could you share with me your wandb account username? I will add you to the openrlbenchmark wandb team. |
I will make a test file for that.
Here's my wandb account: https://wandb.ai/51616 |
Thank you @51616 I have added you to the openrlbenchmark team. You might want to run |
@vwxyzjn I already did the pre-commit hooks for that commit but it still gives an error. I think it has something to do with the edit: turned out the test file was not formatted on my side. |
@vwxyzjn I did the benchmarks. Please let me know if you want any specific updates for this pr. |
Thanks for your patience. The results look great. The next step is to add documentation. Could you give the following command a try? It compares jax.scan with the for loop variant and openai/baselines'PPO.
It should generate a figure and tables ( |
edit: I ran the command with a new environment and it worked just fine but I'm not sure if the python>=3.9 requirement is intended. |
Try again |
Yeah I have just made it compatible with python 3.7.1+ |
Thank you for a quick response. I got the report but I'm not sure where to put it. Which specific doc are you referring to? |
consider adding a section in https://github.com/vwxyzjn/cleanrl/blob/master/docs/rl-algorithms/ppo.md like other ppo variants |
I added the documentation. Not sure if I did it right. Please take a look 🙏 |
There was a problem hiding this comment.
Choose a reason for hiding this comment
The reason will be displayed to describe this comment to others. Learn more.
Thanks for adding the docs. I added some docs and feedback.
docs/rl-algorithms/ppo.md
Outdated
???+ info | ||
|
||
The speed of this variant and [ppo_atari_envpool_xla_jax.py](https://github.com/vwxyzjn/cleanrl/blob/master/cleanrl/ppo_atari_envpool_xla_jax.py) are very similar but the compilation time is reduced significantly (see [vwxyzjn/cleanrl#328](https://github.com/vwxyzjn/cleanrl/pull/328#issuecomment-1340474894)). In the following learning curve, the speed increase comes from the fact that better hardware were used. | ||
|
||
Learning curves: | ||
|
||
 |
There was a problem hiding this comment.
Choose a reason for hiding this comment
The reason will be displayed to describe this comment to others. Learn more.
Yeah, the hardware is a bit of an issue. Would you mind doing two additional things?
1 ) run the ppo_atari_envpool_xla_jax.py
at your machine to align the hardware settings:
export WANDB_ENTITY=openrlbenchmark
python -m cleanrl_utils.benchmark \
--env-ids Pong-v5 BeamRider-v5 Breakout-v5 \
--command "poetry run python cleanrl/ppo_atari_envpool_xla_jax.py --track --capture-video" \
--num-seeds 3 \
--workers 1
- regenerate the learning curves using
openrlbenchmark==0.1.1a3
, which also generates the curves with time as the x-axis. Then you can compare the experiments via
pip install --upgrade openrlbenchmark
python -m openrlbenchmark.rlops \
--filters '?we=openrlbenchmark&wpn=cleanrl&ceik=env_id&cen=exp_name&metric=charts/avg_episodic_return' 'ppo_atari_envpool_xla_jax_scan?tag=pr-328&user=51616' 'ppo_atari_envpool_xla_jax?tag=pr-328&user=51616' \
--filters '?we=openrlbenchmark&wpn=baselines&ceik=env&cen=exp_name&metric=charts/episodic_return' 'baselines-ppo2-cnn' \
--filters '?we=openrlbenchmark&wpn=envpool-atari&ceik=env_id&cen=exp_name&metric=charts/avg_episodic_return' 'ppo_atari_envpool_xla_jax_truncation?user=costa-huang' \
--env-ids BeamRider-v5 Breakout-v5 Pong-v5 \
--check-empty-runs False \
--ncols 3 \
--ncols-legend 2 \
--output-filename compare \
--scan-history \
--report
and maybe in the docs we can specify the hardware differences used by costa-huang
and 51616
:)
There was a problem hiding this comment.
Choose a reason for hiding this comment
The reason will be displayed to describe this comment to others. Learn more.
For some reason, the runs with the python loops didn't get the pr-328
tag. So this filter doesn't work
--filters '?we=openrlbenchmark&wpn=cleanrl&ceik=env_id&cen=exp_name&metric=charts/avg_episodic_return' 'ppo_atari_envpool_xla_jax_scan?tag=pr-328&user=51616' 'ppo_atari_envpool_xla_jax?tag=pr-328&user=51616'
It gives the following error:
{
│ 'wandb_project_name': 'cleanrl',
│ 'wandb_entity': 'openrlbenchmark',
│ 'custom_env_id_key': 'env_id',
│ 'custom_exp_name': 'exp_name',
│ 'metric': 'charts/avg_episodic_return'
}
========= ppo_atari_envpool_xla_jax_scan?tag=pr-328&user=51616
========= ppo_atari_envpool_xla_jax?tag=pr-328&user=51616
{
│ 'wandb_project_name': 'baselines',
│ 'wandb_entity': 'openrlbenchmark',
│ 'custom_env_id_key': 'env',
│ 'custom_exp_name': 'exp_name',
│ 'metric': 'charts/episodic_return'
}
========= baselines-ppo2-cnn
{
│ 'wandb_project_name': 'envpool-atari',
│ 'wandb_entity': 'openrlbenchmark',
│ 'custom_env_id_key': 'env_id',
│ 'custom_exp_name': 'exp_name',
│ 'metric': 'charts/avg_episodic_return'
}
========= ppo_atari_envpool_xla_jax_truncation?user=costa-huang
Traceback (most recent call last):
File "/home/tan/miniconda3/envs/cleanrl/lib/python3.7/runpy.py", line 193, in _run_module_as_main
"__main__", mod_spec)
File "/home/tan/miniconda3/envs/cleanrl/lib/python3.7/runpy.py", line 85, in _run_code
exec(code, run_globals)
File "/home/tan/miniconda3/envs/cleanrl/lib/python3.7/site-packages/openrlbenchmark/rlops.py", line 356, in <module>
time_unit=args.time_unit,
File "/home/tan/miniconda3/envs/cleanrl/lib/python3.7/site-packages/openrlbenchmark/rlops.py", line 161, in compare
{(runsets[idx].report_runset.name, runsets[idx].runs[0].config[runsets[idx].exp_name]): runsets[idx].color}
File "/home/tan/miniconda3/envs/cleanrl/lib/python3.7/site-packages/wandb/apis/public.py", line 1053, in __getitem__
return self.objects[index]
IndexError: list index out of range
Not sure if this is an intended behavior. Anyway, I manually added the tag and the command ran just fine.
There was a problem hiding this comment.
Choose a reason for hiding this comment
The reason will be displayed to describe this comment to others. Learn more.
Hmm that was weird... The cleanrl_utils.benchmark
utility should have autotagged.
There was a problem hiding this comment.
Choose a reason for hiding this comment
The reason will be displayed to describe this comment to others. Learn more.
docs updated
There was a problem hiding this comment.
Choose a reason for hiding this comment
The reason will be displayed to describe this comment to others. Learn more.
There was a problem hiding this comment.
Choose a reason for hiding this comment
The reason will be displayed to describe this comment to others. Learn more.
My cpu is Ryzen 5950x. Maybe that’s factor too.
There was a problem hiding this comment.
Choose a reason for hiding this comment
The reason will be displayed to describe this comment to others. Learn more.
Great job! LGTM. Feel free to merge when you are ready. You should have the contributor's access (merge access) by now. Thanks again.
Description
Modifying the code to use
jax.lax.scan
for fast compile time and small speed improvement.The loss metrics of this pull request (blue) are consistent with the original version (green).
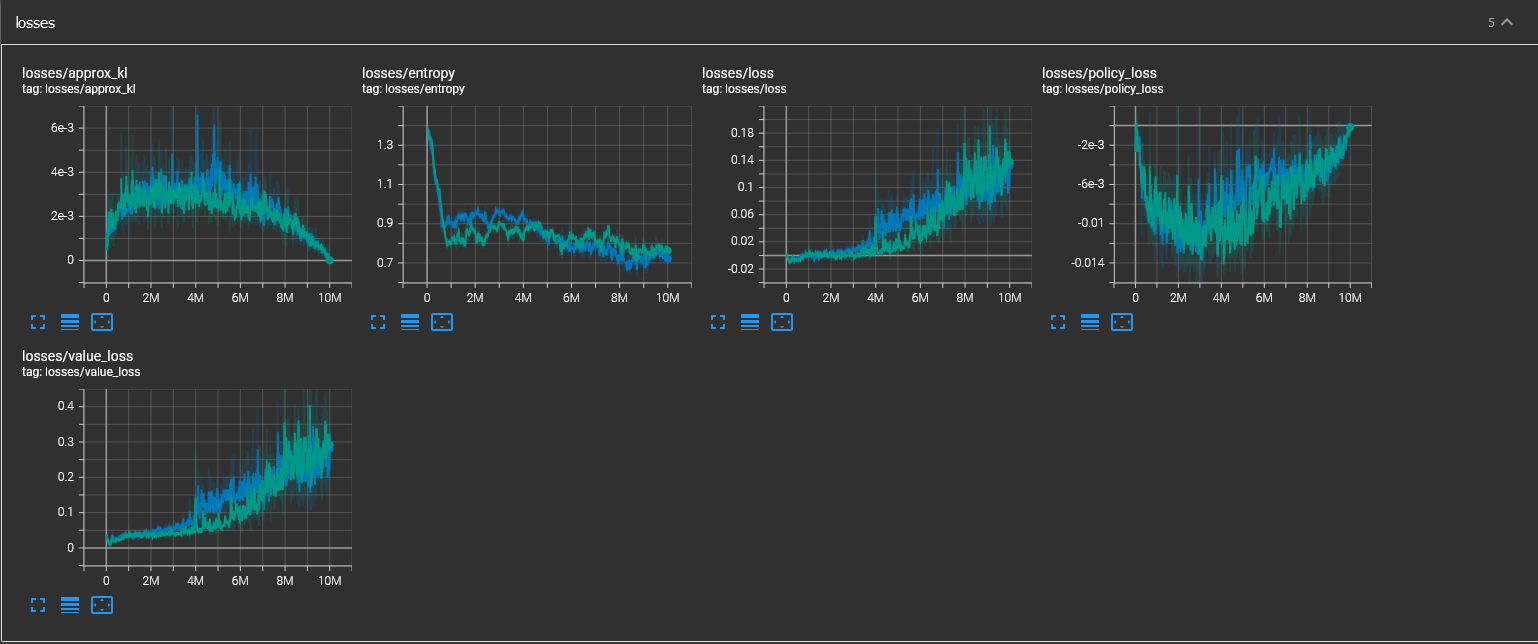
The performance is similar to the original with a slight speed improvement.
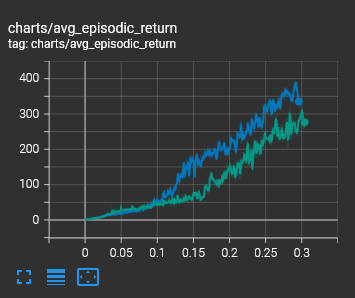
The command used is
python cleanrl/ppo_atari_envpool_xla_jax_scan.py --env-id Breakout-v5 --total-timesteps 10000000 --num-envs 32 --seed 111
(blue) andpython cleanrl/ppo_atari_envpool_xla_jax.py --env-id Breakout-v5 --total-timesteps 10000000 --num-envs 32 --seed 111
(green).Types of changes
Checklist:
pre-commit run --all-files
passes (required).mkdocs serve
.If you are adding new algorithm variants or your change could result in performance difference, you may need to (re-)run tracked experiments. See #137 as an example PR.
--capture-video
flag toggled on (required).mkdocs serve
.