You signed in with another tab or window. Reload to refresh your session.You signed out in another tab or window. Reload to refresh your session.You switched accounts on another tab or window. Reload to refresh your session.Dismiss alert
* Network Analysis: graph centrality and visualization.
10
15
11
-
It is well documented and bundled with 50+ examples and 350+ unit tests. The source code is licensed under BSD and available from <http://www.clips.ua.ac.be/pages/pattern>.
16
+
It is well documented, thoroughly tested with 350+ unit tests and comes bundled with 50+ examples. The source code is licensed under BSD and available from <http://www.clips.ua.ac.be/pages/pattern>.
12
17
13
-
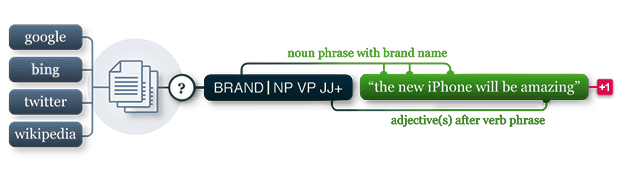
This example trains a classifier on adjectives mined from Twitter using Python 3. First, tweets that contain hashtag #win or #fail are collected. For example: *"$20 tip off a sweet little old lady today #win"*. The word part-of-speech tags are then parsed, keeping only adjectives. Each tweet is transformed to a vector, a dictionary of adjective → count items, labeled `WIN` or `FAIL`. The classifier uses the vectors to learn which other tweets look more like `WIN` or more like `FAIL`.
19
24
20
-
License
21
-
-------
25
+
```python
26
+
from pattern.web import Twitter
27
+
from pattern.en import tag
28
+
from pattern.vector importKNN, count
22
29
23
-
**BSD**, see `LICENSE.txt` for further details.
30
+
twitter, knn = Twitter(), KNN()
31
+
32
+
for i inrange(1, 3):
33
+
for tweet in twitter.search('#win OR #fail', start=i, count=100):
34
+
s = tweet.text.lower()
35
+
p ='#win'in s and'WIN'or'FAIL'
36
+
v = tag(s)
37
+
v = [word for word, pos in v if pos =='JJ'] # JJ = adjective
38
+
v = count(v) # {'sweet': 1}
39
+
if v:
40
+
knn.train(v, type=p)
41
+
42
+
print(knn.classify('sweet potato burger'))
43
+
print(knn.classify('stupid autocorrect'))
44
+
```
24
45
25
46
Installation
26
47
------------
27
48
28
-
Pattern is written for Python 2.5+ (no support for Python 3 yet). The module has no external dependencies except when using LSA in the pattern.vector module, which requires NumPy (installed by default on Mac OS X). To install Pattern so that it is available in all your scripts, unzip the download and from the command line do:
49
+
Pattern supports Python 2.7 and Python 3.6+. The Python 3 version is currently **only** available on the `development` branch. To install Pattern so that it is available in all your scripts, unzip the download and from the command line do:
29
50
```bash
30
51
cd pattern-2.6
31
52
python setup.py install
32
53
```
33
54
34
-
If you have pip, you can automatically download and install from the PyPi repository:
55
+
If you have pip, you can automatically download and install from the [PyPI repository](https://pypi.python.org/pypi/Pattern):
35
56
```bash
36
57
pip install pattern
37
58
```
@@ -50,36 +71,20 @@ import sys; if MODULE not in sys.path: sys.path.append(MODULE)
50
71
from pattern.en import parsetree
51
72
```
52
73
53
-
Example
54
-
-------
55
-
56
-
This example trains a classifier on adjectives mined from Twitter. First, tweets that contain hashtag #win or #fail are collected. For example: "$20 tip off a sweet little old lady today #win". The word part-of-speech tags are then parsed, keeping only adjectives. Each tweet is transformed to a vector, a dictionary of adjective → count items, labeled `WIN` or `FAIL`. The classifier uses the vectors to learn which other tweets look more like `WIN` or more like `FAIL`.
57
-
58
-
```python
59
-
from pattern.web import Twitter
60
-
from pattern.en import tag
61
-
from pattern.vector importKNN, count
74
+
Documentation
75
+
-------------
62
76
63
-
twitter, knn = Twitter(), KNN()
77
+
For documentation and examples see the [user documentation](http://www.clips.ua.ac.be/pages/pattern). If you are a developer, go check out the [developer documentation](http://www.clips.ua.ac.be/pages/pattern-dev).
64
78
65
-
for i inrange(1, 3):
66
-
for tweet in twitter.search('#win OR #fail', start=i, count=100):
67
-
s = tweet.text.lower()
68
-
p ='#win'in s and'WIN'or'FAIL'
69
-
v = tag(s)
70
-
v = [word for word, pos in v if pos =='JJ'] # JJ = adjective
71
-
v = count(v) # {'sweet': 1}
72
-
if v:
73
-
knn.train(v, type=p)
79
+
Version
80
+
-------
74
81
75
-
print knn.classify('sweet potato burger')
76
-
print knn.classify('stupid autocorrect')
77
-
```
82
+
2.6
78
83
79
-
Documentation
80
-
-------------
84
+
License
85
+
-------
81
86
82
-
<http://www.clips.ua.ac.be/pages/pattern>
87
+
**BSD**, see `LICENSE.txt` for further details.
83
88
84
89
Reference
85
90
---------
@@ -89,14 +94,13 @@ De Smedt, T., Daelemans, W. (2012). Pattern for Python. *Journal of Machine Lear
89
94
Contribute
90
95
----------
91
96
92
-
The source code is hosted on GitHub and contributions or donations are welcomed, see the [developer documentation](http://www.clips.ua.ac.be/pages/pattern#contribute). If you use Pattern in your work, please cite our reference paper.
97
+
The source code is hosted on GitHub and contributions or donations are welcomed. Please have look at the [developer documentation](http://www.clips.ua.ac.be/pages/pattern-dev). If you use Pattern in your work, please cite our reference paper.
93
98
94
99
Bundled dependencies
95
100
--------------------
96
101
97
102
Pattern is bundled with the following data sets, algorithms and Python packages:
98
103
99
-
-**Beautiful Soup**, Leonard Richardson
100
104
-**Brill tagger**, Eric Brill
101
105
-**Brill tagger for Dutch**, Jeroen Geertzen
102
106
-**Brill tagger for German**, Gerold Schneider & Martin Volk
@@ -110,13 +114,7 @@ Pattern is bundled with the following data sets, algorithms and Python packages:
110
114
-**LIBSVM**, Chih-Chung Chang & Chih-Jen Lin
111
115
-**LIBLINEAR**, Rong-En Fan et al.
112
116
-**NetworkX centrality**, Aric Hagberg, Dan Schult & Pieter Swart
0 commit comments