Commit 38d58ba
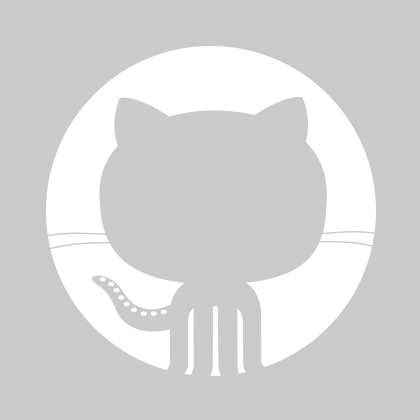
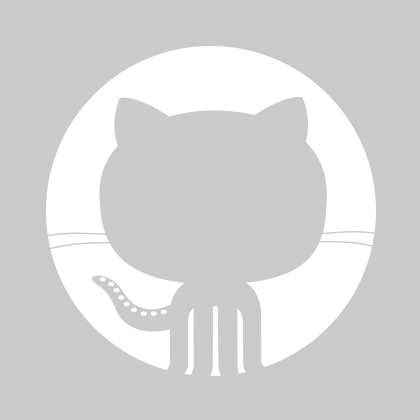
Christopher B. Choy
Christopher B. Choy
1 parent 9953665 commit 38d58ba
3 files changed
+8
-5
lines changed+8-5
Original file line number | Diff line number | Diff line change | |
---|---|---|---|
| |||
22 | 22 |
| |
23 | 23 |
| |
24 | 24 |
| |
25 |
| - | |
26 |
| - | |
27 |
| - | |
| 25 | + | |
| 26 | + | |
| 27 | + | |
28 | 28 |
| |
29 |
| - | |
| 29 | + | |
| 30 | + | |
| 31 | + | |
| 32 | + | |
30 | 33 |
| |
31 | 34 |
| |
32 | 35 |
| |
| |||
111 | 114 |
| |
112 | 115 |
| |
113 | 116 |
| |
114 |
| - | |
| 117 | + | |
115 | 118 |
| |
116 | 119 |
|
121 KB
Loading
31.3 KB
Loading
0 commit comments