diff --git a/docs/python_docs/python/tutorials/packages/autograd/_static/autograd_control_flow.png b/docs/python_docs/_static/autograd_images/autograd_control_flow.png
similarity index 100%
rename from docs/python_docs/python/tutorials/packages/autograd/_static/autograd_control_flow.png
rename to docs/python_docs/_static/autograd_images/autograd_control_flow.png
diff --git a/docs/python_docs/python/tutorials/packages/autograd/_static/autograd_control_flow_grad.png b/docs/python_docs/_static/autograd_images/autograd_control_flow_grad.png
similarity index 100%
rename from docs/python_docs/python/tutorials/packages/autograd/_static/autograd_control_flow_grad.png
rename to docs/python_docs/_static/autograd_images/autograd_control_flow_grad.png
diff --git a/docs/python_docs/python/tutorials/packages/autograd/_static/autograd_forward_backward.png b/docs/python_docs/_static/autograd_images/autograd_forward_backward.png
similarity index 100%
rename from docs/python_docs/python/tutorials/packages/autograd/_static/autograd_forward_backward.png
rename to docs/python_docs/_static/autograd_images/autograd_forward_backward.png
diff --git a/docs/python_docs/python/tutorials/packages/autograd/_static/autograd_grad_req.mp4 b/docs/python_docs/_static/autograd_images/autograd_grad_req.mp4
similarity index 100%
rename from docs/python_docs/python/tutorials/packages/autograd/_static/autograd_grad_req.mp4
rename to docs/python_docs/_static/autograd_images/autograd_grad_req.mp4
diff --git a/docs/python_docs/python/tutorials/packages/autograd/_static/autograd_gradient.png b/docs/python_docs/_static/autograd_images/autograd_gradient.png
similarity index 100%
rename from docs/python_docs/python/tutorials/packages/autograd/_static/autograd_gradient.png
rename to docs/python_docs/_static/autograd_images/autograd_gradient.png
diff --git a/docs/python_docs/python/tutorials/packages/autograd/_static/autograd_graph.mp4 b/docs/python_docs/_static/autograd_images/autograd_graph.mp4
similarity index 100%
rename from docs/python_docs/python/tutorials/packages/autograd/_static/autograd_graph.mp4
rename to docs/python_docs/_static/autograd_images/autograd_graph.mp4
diff --git a/docs/python_docs/python/tutorials/packages/autograd/_static/autograd_head_grad.mp4 b/docs/python_docs/_static/autograd_images/autograd_head_grad.mp4
similarity index 100%
rename from docs/python_docs/python/tutorials/packages/autograd/_static/autograd_head_grad.mp4
rename to docs/python_docs/_static/autograd_images/autograd_head_grad.mp4
diff --git a/docs/python_docs/python/tutorials/deploy/index.rst b/docs/python_docs/python/tutorials/deploy/index.rst
index e0ff0db75f17..9c4d60578226 100644
--- a/docs/python_docs/python/tutorials/deploy/index.rst
+++ b/docs/python_docs/python/tutorials/deploy/index.rst
@@ -29,8 +29,9 @@ The following tutorials will help you learn export MXNet models.
.. card::
:title: Export ONNX Models
+ :link: export/onnx.html
- COMING SOON
+ Export your MXNet model to the Open Neural Exchange Format
.. card::
:title: Export with GluonCV
diff --git a/docs/python_docs/python/tutorials/packages/autograd/index.md b/docs/python_docs/python/tutorials/packages/autograd/index.md
index 6623e15c1e2b..b1fe274ec41f 100644
--- a/docs/python_docs/python/tutorials/packages/autograd/index.md
+++ b/docs/python_docs/python/tutorials/packages/autograd/index.md
@@ -23,7 +23,7 @@
Gradients are fundamental to the process of training neural networks, and tell us how to change the parameters of the network to improve its performance.
-
+
### Long Answer:
@@ -39,7 +39,7 @@ Assuming we've calculated the gradient of each parameter with respect to the los
We differentiate. [MXNet Gluon](/api/python/docs/tutorials/packages/gluon/index.html) uses Reverse Mode Automatic Differentiation (`autograd`) to backprogate gradients from the loss metric to the network parameters.
-
+
### Long Answer:
@@ -56,7 +56,7 @@ Stage 2. Work backwards through this record and evaluate the partial derivatives
@@ -217,7 +217,7 @@ def f(x):
We can plot the resultant function for $x$ between 0 and 1, and we should recognise certain functions in segments of $x$. Starting with a quadratic curve from 0 to 1/2, we have a cubic curve from 1/2 to 2/3, a quartic from 2/3 to 3/4 and finally a flatline.
-
+
Using `autograd`, let's now find the gradient of this arbritrary function. We don't have a vectorized function in this case, because of the control flow, so let's also create a function to calculate the gradient using `autograd`.
@@ -234,7 +234,7 @@ grads = [get_grad(f, x).asscalar() for x in xs]
print(grads)
```
-
+
We can calculate the gradients by hand in this situation (since it's a toy example), and for the four segments discussed before we'd expect $2x$, $3x^2$, $4x^3$ and 0. As a spot check, for $x=0.6$ the hand calculated gradient would be $3x^2=1.08$, which equals `1.08` as computed by `autograd`.
@@ -247,7 +247,7 @@ Most of the time `autograd` will be aware of the complete computational graph, a
diff --git a/docs/python_docs/python/tutorials/packages/gluon/data/data_augmentation.md b/docs/python_docs/python/tutorials/packages/gluon/data/data_augmentation.md
new file mode 100644
index 000000000000..0e320fc2890e
--- /dev/null
+++ b/docs/python_docs/python/tutorials/packages/gluon/data/data_augmentation.md
@@ -0,0 +1,235 @@
+
+
+
+
+
+
+
+
+
+
+
+
+
+
+
+
+
+# Image Augmentation
+
+Augmentation is the process of randomly adjusting the dataset samples used for training. As a result, a greater diversity of samples will be seen by the network and it is therefore less likely to overfit the training dataset. Some of the spurious characteristics of the dataset can be reduced using this technique. One example would be a dataset of images from the same camera having the same color tint: it's unhelpful when you want to apply this model to images from other cameras. You can avoid this by randomly shifting the colours of each image slightly and training your network on these augmented images.
+
+Although this technique can be applied in a variety of domains, it's very common in Computer Vision, and we will focus on image augmentations in this tutorial. Some example image augmentations include random crops and flips, and adjustments to the brightness and contrast.
+
+#### What are the prerequisites?
+
+You should be familiar with the concept of a transform and how to apply it to a dataset before reading this tutorial. Check out the [Data Transforms tutorial]() if this is new to you or you need a quick refresher.
+
+#### Where can I find the augmentation transforms?
+
+You can find them in the `mxnet.gluon.data.vision.transforms` module, alongside the deterministic transforms we've seen previously, such as `ToTensor`, `Normalize`, `CenterCrop` and `Resize`. Augmentations involve an element of randomness and all the augmentation transforms are prefixed with `Random`, such as `RandomResizedCrop` and `RandomBrightness`. We'll start by importing MXNet and the `transforms`.
+
+
+```python
+import matplotlib.pyplot as plt
+import mxnet as mx
+from mxnet.gluon.data.vision import transforms
+```
+
+#### Sample Image
+
+So that we can see the effects of all the vision augmentations, we'll take a sample image of a giraffe and apply various augmentations to it. We can see what it looks like to begin with.
+
+
+```python
+image_url = 'https://raw.githubusercontent.com/dmlc/web-data/master/mxnet/doc/tutorials/data_aug/inputs/0.jpg'
+mx.test_utils.download(image_url, "giraffe.jpg")
+example_image = mx.image.imread("giraffe.jpg")
+plt.imshow(example_image.asnumpy())
+```
+
+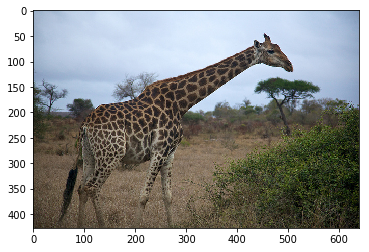
+
+
+Since these augmentations are random, we'll apply the same augmentation a few times and plot all of the outputs. We define a few utility functions to help with this.
+
+
+```python
+def show_images(imgs, num_rows, num_cols, scale=2):
+ # show augmented images in a grid layout
+ aspect_ratio = imgs[0].shape[0]/imgs[0].shape[1]
+ figsize = (num_cols * scale, num_rows * scale * aspect_ratio)
+ _, axes = plt.subplots(num_rows, num_cols, figsize=figsize)
+ for i in range(num_rows):
+ for j in range(num_cols):
+ axes[i][j].imshow(imgs[i * num_cols + j].asnumpy())
+ axes[i][j].axes.get_xaxis().set_visible(False)
+ axes[i][j].axes.get_yaxis().set_visible(False)
+ plt.subplots_adjust(hspace=0.1, wspace=0)
+ return axes
+
+def apply(img, aug, num_rows=2, num_cols=4, scale=3):
+ # apply augmentation multiple times to obtain different samples
+ Y = [aug(img) for _ in range(num_rows * num_cols)]
+ show_images(Y, num_rows, num_cols, scale)
+```
+
+# Spatial Augmentation
+
+One form of augmentation affects the spatial position of pixel values. Using combinations of slicing, scaling, translating, rotating and flipping the values of the original image can be shifted to create new images. Some operations (like scaling and rotation) require interpolation as pixels in the new image are combinations of pixels in the original image.
+
+### `RandomResizedCrop`
+
+Many Computer Visions tasks, such as image classification and object detection, should be robust to changes in the scale and position of objects in the image. You can incorporate this into the network using pooling layers, but an alternative method is to crop random regions of the original image.
+
+As an example, we randomly (using a uniform distribution) crop a region of the image with:
+
+* an area of 10% to 100% of the original area
+* a ratio of width to height between 0.5 and 2
+
+And then we resize this cropped region to 200 by 200 pixels.
+
+
+```python
+shape_aug = transforms.RandomResizedCrop(size=(200, 200),
+ scale=(0.1, 1),
+ ratio=(0.5, 2))
+apply(example_image, shape_aug)
+```
+
+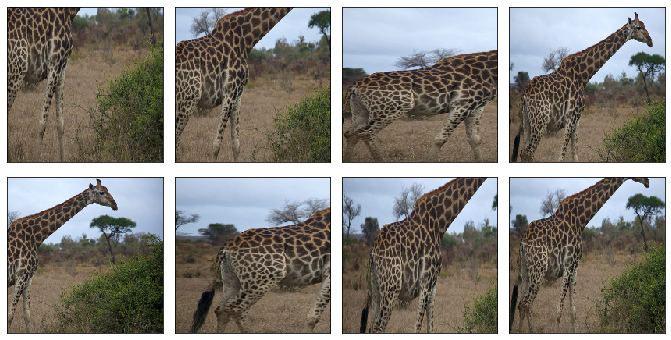
+
+
+### `RandomFlipLeftRight`
+
+A simple augmentation technique is flipping. Usually flipping horizontally doesn't change the category of object and results in an image that's still plausible in the real world. Using `RandomFlipLeftRight`, we randomly flip the image horizontally 50% of the time.
+
+
+```python
+apply(example_image, transforms.RandomFlipLeftRight())
+```
+
+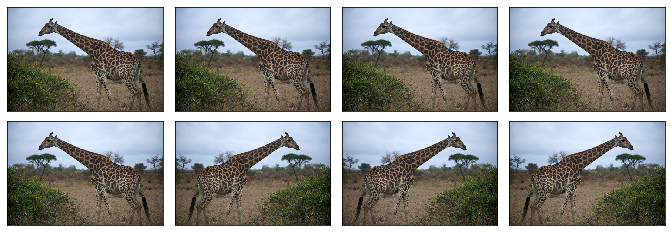
+
+
+### `RandomFlipTopBottom`
+
+Although it's not as common as flipping left and right, you can flip the image vertically 50% of the time with `RandomFlipTopBottom`. With our giraffe example, we end up with less plausible samples that horizontal flipping, with the ground above the sky in some cases.
+
+
+```python
+apply(example_image, transforms.RandomFlipTopBottom())
+```
+
+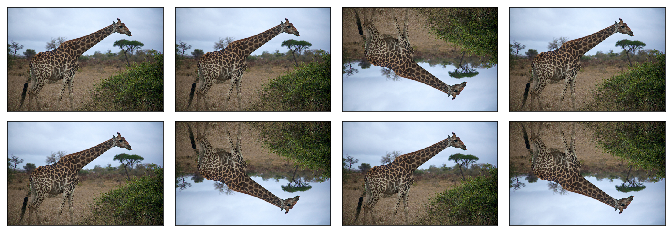
+
+
+# Color Augmentation
+
+Usually, exact coloring doesn't play a significant role in the classification or detection of objects, so augmenting the colors of images is a good technique to make the network invariant to color shifts. Color properties that can be changed include brightness, contrast, saturation and hue.
+
+### `RandomBrightness`
+
+Use `RandomBrightness` to add a random brightness jitter to images. Use the `brightness` parameter to control the amount of jitter in brightness, with value from 0 (no change) to 1 (potentially large change). `brightness` doesn't specify whether the brightness of the augmented image will be lighter or darker, just the potential strength of the effect. Specifically the augmentation is given by:
+
+```
+alpha = 1.0 + random.uniform(-brightness, brightness)
+image *= alpha
+```
+
+So by setting this to 0.5 we randomly change the brightness of the image to a value between 50% ($1-0.5$) and 150% ($1+0.5$) of the original image.
+
+
+```python
+apply(example_image, transforms.RandomBrightness(0.5))
+```
+
+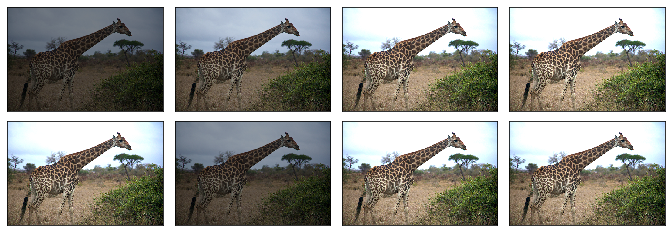
+
+
+### `RandomContrast`
+
+Use `RandomContrast` to add a random contrast jitter to an image. Contrast can be thought of as the degree to which light and dark colors in the image differ. Use the `contrast` parameter to control the amount of jitter in contrast, with value from 0 (no change) to 1 (potentially large change). `contrast` doesn't specify whether the contrast of the augmented image will be higher or lower, just the potential strength of the effect. Specifically, the augmentation is given by:
+
+```
+coef = nd.array([[[0.299, 0.587, 0.114]]])
+alpha = 1.0 + random.uniform(-contrast, contrast)
+gray = image * coef
+gray = (3.0 * (1.0 - alpha) / gray.size) * nd.sum(gray)
+image *= alpha
+image += gray
+```
+
+
+```python
+apply(example_image, transforms.RandomContrast(0.5))
+```
+
+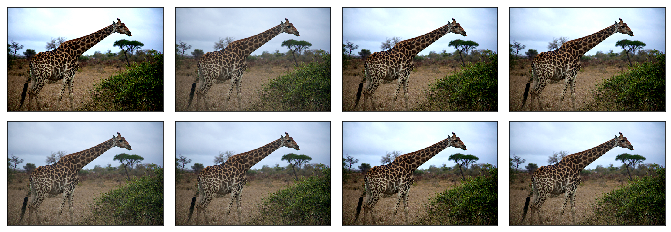
+
+
+### `RandomSaturation`
+
+Use `RandomSaturation` to add a random saturation jitter to an image. Saturation can be thought of as the 'amount' of color in an image. Use the `saturation` parameter to control the amount of jitter in saturation, with value from 0 (no change) to 1 (potentially large change). `saturation` doesn't specify whether the saturation of the augmented image will be higher or lower, just the potential strength of the effect. Specifically the augmentation is using the method detailed [here](https://beesbuzz.biz/code/16-hsv-color-transforms).
+
+
+```python
+apply(example_image, transforms.RandomSaturation(0.5))
+```
+
+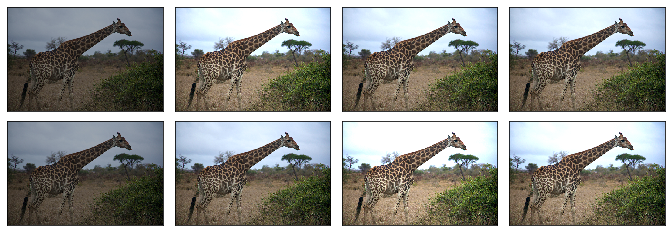
+
+
+### `RandomHue`
+
+Use `RandomHue` to add a random hue jitter to images. Hue can be thought of as the 'shade' of the colors in an image. Use the `hue` parameter to control the amount of jitter in hue, with value from 0 (no change) to 1 (potentially large change). `hue` doesn't specify whether the hue of the augmented image will be shifted one way or the other, just the potential strength of the effect. Specifically the augmentation is using the method detailed [here](https://beesbuzz.biz/code/16-hsv-color-transforms).
+
+
+```python
+apply(example_image, transforms.RandomHue(0.5))
+```
+
+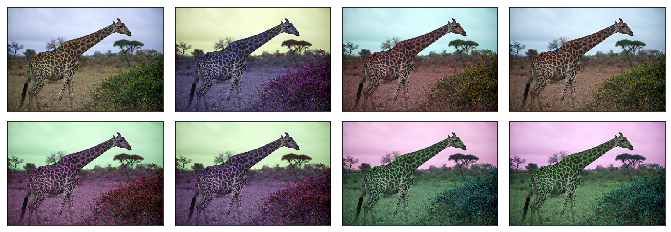
+
+
+### `RandomColorJitter`
+
+`RandomColorJitter` is a convenience transform that can be used to perform multiple color augmentations at once. You can set the `brightness`, `contrast`, `saturation` and `hue` jitters, that function the same as above for their individual transforms.
+
+
+```python
+color_aug = transforms.RandomColorJitter(brightness=0.5,
+ contrast=0.5,
+ saturation=0.5,
+ hue=0.5)
+apply(example_image, color_aug)
+```
+
+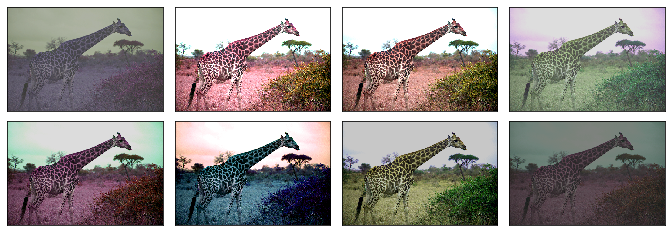
+
+
+### `RandomLighting`
+
+Use `RandomLighting` for an AlexNet-style PCA-based noise augmentation.
+
+
+```python
+apply(example_image, transforms.RandomLighting(alpha=1))
+```
+
+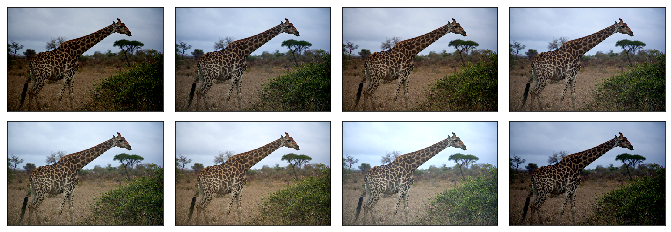
+
+# Composed Augmentations
+
+In practice, we apply multiple augmentation techniques to an image to increase the variety of images in the dataset. Using the `Compose` transform that was introduced in the [Data Transforms tutorial](), we can apply 3 of the transforms we previously used above.
+
+
+```python
+augs = transforms.Compose([
+ transforms.RandomFlipLeftRight(), color_aug, shape_aug])
+apply(example_image, augs)
+```
+
+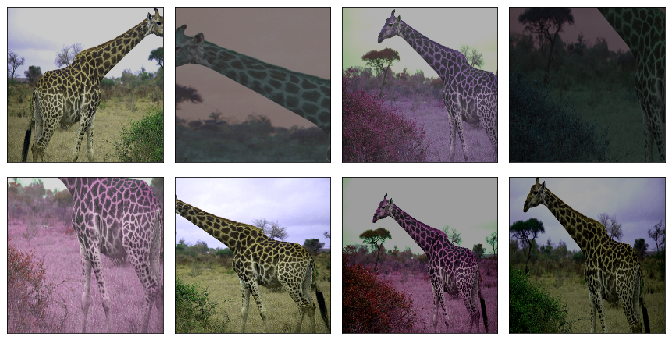
+
+
\ No newline at end of file
diff --git a/docs/python_docs/python/tutorials/packages/gluon/data/datasets.md b/docs/python_docs/python/tutorials/packages/gluon/data/datasets.md
new file mode 100644
index 000000000000..1c952b86a3da
--- /dev/null
+++ b/docs/python_docs/python/tutorials/packages/gluon/data/datasets.md
@@ -0,0 +1,333 @@
+
+
+
+
+
+
+
+
+
+
+
+
+
+
+
+
+
+
+# Gluon `Dataset`s and `DataLoader`
+
+One of the most critical steps for model training and inference is loading the data: without data you can't do Machine Learning! In this tutorial we use the Gluon API to define a [`Dataset`](/api/python/gluon/data.html?highlight=dataset#mxnet.gluon.data.Dataset) and use a [`DataLoader`](/api/python/gluon/data.html?highlight=dataloader#mxnet.gluon.data.DataLoader) to iterate through the dataset in mini-batches.
+
+## Introduction to `Dataset`s
+
+[`Dataset`](/api/python/gluon/data.html?highlight=dataset#mxnet.gluon.data.Dataset) objects are used to represent collections of data, and include methods to load and parse the data (that is often stored on disk). Gluon has a number of different [`Dataset`](/api/python/gluon/data.html?highlight=dataset#mxnet.gluon.data.Dataset) classes for working with image data straight out-of-the-box, but we'll use the [`ArrayDataset`](/api/python/gluon/data.html) to introduce the idea of a [`Dataset`](/api/python/gluon/data.html?highlight=dataset#mxnet.gluon.data.Dataset).
+
+We first start by generating random data `X` (with 3 variables) and corresponding random labels `y` to simulate a typical supervised learning task. We generate 10 samples and we pass them all to the [`ArrayDataset`](/api/python/gluon/data/data.html).
+
+
+```python
+import mxnet as mx
+import os
+import tarfile
+
+mx.random.seed(42) # Fix the seed for reproducibility
+X = mx.random.uniform(shape=(10, 3))
+y = mx.random.uniform(shape=(10, 1))
+dataset = mx.gluon.data.dataset.ArrayDataset(X, y)
+```
+
+A key feature of a [`Dataset`](https://mxnet.incubator.apache.org/api/python/gluon/data.html?highlight=dataset#mxnet.gluon.data.Dataset) is the __*ability to retrieve a single sample given an index*__. Our random data and labels were generated in memory, so this [`ArrayDataset`](https://mxnet.incubator.apache.org/api/python/gluon/data.html?highlight=arraydataset#mxnet.gluon.data.ArrayDataset) doesn't have to load anything from disk, but the interface is the same for all [`Dataset`](https://mxnet.incubator.apache.org/api/python/gluon/data.html?highlight=dataset#mxnet.gluon.data.Dataset)s.
+
+
+```python
+sample_idx = 4
+sample = dataset[sample_idx]
+
+assert len(sample) == 2
+assert sample[0].shape == (3, )
+assert sample[1].shape == (1, )
+print(sample)
+```
+
+(
+[ 0.4375872 0.29753461 0.89177299]
+,
+[ 0.83261985]
+)
+
+
+We get a tuple of a data sample and its corresponding label, which makes sense because we passed the data `X` and the labels `y` in that order when we instantiated the [`ArrayDataset`](https://mxnet.incubator.apache.org/api/python/gluon/data.html?highlight=arraydataset#mxnet.gluon.data.ArrayDataset). We don't usually retrieve individual samples from [`Dataset`](https://mxnet.incubator.apache.org/api/python/gluon/data.html?highlight=dataset#mxnet.gluon.data.Dataset) objects though (unless we're quality checking the output samples). Instead we use a [`DataLoader`](https://mxnet.incubator.apache.org/api/python/gluon/data.html?highlight=dataloader#mxnet.gluon.data.DataLoader).
+
+## Introduction to `DataLoader`
+
+A [`DataLoader`](https://mxnet.incubator.apache.org/api/python/gluon/data.html?highlight=dataloader#mxnet.gluon.data.DataLoader) is used to create mini-batches of samples from a [`Dataset`](https://mxnet.incubator.apache.org/api/python/gluon/data.html?highlight=dataset#mxnet.gluon.data.Dataset), and provides a convenient iterator interface for looping these batches. It's typically much more efficient to pass a mini-batch of data through a neural network than a single sample at a time, because the computation can be performed in parallel. A required parameter of [`DataLoader`](https://mxnet.incubator.apache.org/api/python/gluon/data.html?highlight=dataloader#mxnet.gluon.data.DataLoader) is the size of the mini-batches you want to create, called `batch_size`.
+
+Another benefit of using [`DataLoader`](https://mxnet.incubator.apache.org/api/python/gluon/data.html?highlight=dataloader#mxnet.gluon.data.DataLoader) is the ability to easily load data in parallel using [`multiprocessing`](https://docs.python.org/3.6/library/multiprocessing.html). You can set the `num_workers` parameter to the number of CPUs avalaible on your machine for maximum performance, or limit it to a lower number to spare resources.
+
+
+```python
+from multiprocessing import cpu_count
+CPU_COUNT = cpu_count()
+
+data_loader = mx.gluon.data.DataLoader(dataset, batch_size=5, num_workers=CPU_COUNT)
+
+for X_batch, y_batch in data_loader:
+ print("X_batch has shape {}, and y_batch has shape {}".format(X_batch.shape, y_batch.shape))
+```
+
+`X_batch has shape (5, 3), and y_batch has shape (5, 1)`
+
+`X_batch has shape (5, 3), and y_batch has shape (5, 1)`
+
+
+We can see 2 mini-batches of data (and labels), each with 5 samples, which makes sense given we started with a dataset of 10 samples. When comparing the shape of the batches to the samples returned by the [`Dataset`](https://mxnet.incubator.apache.org/api/python/gluon/data.html?highlight=dataset#mxnet.gluon.data.Dataset), we've gained an extra dimension at the start which is sometimes called the batch axis.
+
+Our `data_loader` loop will stop when every sample of `dataset` has been returned as part of a batch. Sometimes the dataset length isn't divisible by the mini-batch size, leaving a final batch with a smaller number of samples. [`DataLoader`](https://mxnet.incubator.apache.org/api/python/gluon/data.html?highlight=dataloader#mxnet.gluon.data.DataLoader)'s default behavior is to return this smaller mini-batch, but this can be changed by setting the `last_batch` parameter to `discard` (which ignores the last batch) or `rollover` (which starts the next epoch with the remaining samples).
+
+## Machine learning with `Dataset`s and `DataLoader`s
+
+You will often use a few different [`Dataset`](https://mxnet.incubator.apache.org/api/python/gluon/data.html?highlight=dataset#mxnet.gluon.data.Dataset) objects in your Machine Learning project. It's essential to separate your training dataset from testing dataset, and it's also good practice to have validation dataset (a.k.a. development dataset) that can be used for optimising hyperparameters.
+
+Using Gluon [`Dataset`](https://mxnet.incubator.apache.org/api/python/gluon/data.html?highlight=dataset#mxnet.gluon.data.Dataset) objects, we define the data to be included in each of these separate datasets. Common use cases for loading data are covered already (e.g. [`mxnet.gluon.data.vision.datasets.ImageFolderDataset`](https://mxnet.incubator.apache.org/api/python/gluon/data.html?highlight=imagefolderdataset#mxnet.gluon.data.vision.datasets.ImageFolderDataset)), but it's simple to create your own custom [`Dataset`](https://mxnet.incubator.apache.org/api/python/gluon/data.html?highlight=dataset#mxnet.gluon.data.Dataset) classes for other types of data. You can even use included [`Dataset`](https://mxnet.incubator.apache.org/api/python/gluon/data.html?highlight=dataset#mxnet.gluon.data.Dataset) objects for common datasets if you want to experiment quickly; they download and parse the data for you! In this example we use the [Fashion MNIST](https://github.com/zalandoresearch/fashion-mnist) dataset from Zalando Research.
+
+Many of the image [`Dataset`](https://mxnet.incubator.apache.org/api/python/gluon/data.html?highlight=dataset#mxnet.gluon.data.Dataset)s accept a function (via the optional `transform` parameter) which is applied to each sample returned by the [`Dataset`](https://mxnet.incubator.apache.org/api/python/gluon/data.html?highlight=dataset#mxnet.gluon.data.Dataset). It's useful for performing data augmentation, but can also be used for more simple data type conversion and pixel value scaling as seen below.
+
+
+```python
+def transform(data, label):
+ data = data.astype('float32')/255
+ return data, label
+
+train_dataset = mx.gluon.data.vision.datasets.FashionMNIST(train=True, transform=transform)
+valid_dataset = mx.gluon.data.vision.datasets.FashionMNIST(train=False, transform=transform)
+```
+
+
+```python
+%matplotlib inline
+from matplotlib.pylab import imshow
+
+sample_idx = 234
+sample = train_dataset[sample_idx]
+data = sample[0]
+label = sample[1]
+label_desc = {0:'T-shirt/top', 1:'Trouser', 2:'Pullover', 3:'Dress', 4:'Coat', 5:'Sandal', 6:'Shirt', 7:'Sneaker', 8:'Bag', 9:'Ankle boot'}
+
+imshow(data[:,:,0].asnumpy(), cmap='gray')
+print("Data type: {}".format(data.dtype))
+print("Label: {}".format(label))
+print("Label description: {}".format(label_desc[label]))
+```
+
+`Data type: `
+
+`Label: 8`
+
+`Label description: Bag`
+
+
+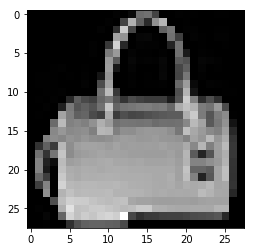
+
+
+When training machine learning models it is important to shuffle the training samples every time you pass through the dataset (i.e. each epoch). Sometimes the order of your samples will have a spurious relationship with the target variable, and shuffling the samples helps remove this. With [`DataLoader`](https://mxnet.incubator.apache.org/api/python/gluon/data.html?highlight=dataloader#mxnet.gluon.data.DataLoader) it's as simple as adding `shuffle=True`. You don't need to shuffle the validation and testing data though.
+
+If you have more complex shuffling requirements (e.g. when handling sequential data), take a look at [`mxnet.gluon.data.BatchSampler`](https://mxnet.incubator.apache.org/api/python/gluon/data.html?highlight=batchsampler#mxnet.gluon.data.BatchSampler) and pass this to your [`DataLoader`](https://mxnet.incubator.apache.org/api/python/gluon/data.html?highlight=dataloader#mxnet.gluon.data.DataLoader) instead.
+
+
+```python
+batch_size = 32
+train_data_loader = mx.gluon.data.DataLoader(train_dataset, batch_size, shuffle=True, num_workers=CPU_COUNT)
+valid_data_loader = mx.gluon.data.DataLoader(valid_dataset, batch_size, num_workers=CPU_COUNT)
+```
+
+With both `DataLoader`s defined, we can now train a model to classify each image and evaluate the validation loss at each epoch. Our Fashion MNIST dataset has 10 classes including shirt, dress, sneakers, etc. We define a simple fully connected network with a softmax output and use cross entropy as our loss.
+
+
+```python
+from mxnet import gluon, autograd, ndarray
+
+def construct_net():
+ net = gluon.nn.HybridSequential()
+ with net.name_scope():
+ net.add(gluon.nn.Dense(128, activation="relu"))
+ net.add(gluon.nn.Dense(64, activation="relu"))
+ net.add(gluon.nn.Dense(10))
+ return net
+
+# construct and initialize network.
+ctx = mx.gpu() if mx.context.num_gpus() else mx.cpu()
+
+net = construct_net()
+net.hybridize()
+net.initialize(mx.init.Xavier(), ctx=ctx)
+# define loss and trainer.
+criterion = gluon.loss.SoftmaxCrossEntropyLoss()
+trainer = gluon.Trainer(net.collect_params(), 'sgd', {'learning_rate': 0.1})
+```
+
+```python
+
+
+epochs = 5
+for epoch in range(epochs):
+ # training loop (with autograd and trainer steps, etc.)
+ cumulative_train_loss = mx.nd.zeros(1, ctx=ctx)
+ training_samples = 0
+ for batch_idx, (data, label) in enumerate(train_data_loader):
+ data = data.as_in_context(ctx).reshape((-1, 784)) # 28*28=784
+ label = label.as_in_context(ctx)
+ with autograd.record():
+ output = net(data)
+ loss = criterion(output, label)
+ loss.backward()
+ trainer.step(data.shape[0])
+ cumulative_train_loss += loss.sum()
+ training_samples += data.shape[0]
+ train_loss = cumulative_train_loss.asscalar()/training_samples
+
+ # validation loop
+ cumulative_valid_loss = mx.nd.zeros(1, ctx)
+ valid_samples = 0
+ for batch_idx, (data, label) in enumerate(valid_data_loader):
+ data = data.as_in_context(ctx).reshape((-1, 784)) # 28*28=784
+ label = label.as_in_context(ctx)
+ output = net(data)
+ loss = criterion(output, label)
+ cumulative_valid_loss += loss.sum()
+ valid_samples += data.shape[0]
+ valid_loss = cumulative_valid_loss.asscalar()/valid_samples
+
+ print("Epoch {}, training loss: {:.2f}, validation loss: {:.2f}".format(epoch, train_loss, valid_loss))
+```
+
+`Epoch 0, training loss: 0.54, validation loss: 0.45`
+
+`...`
+
+`Epoch 4, training loss: 0.32, validation loss: 0.33`
+
+
+# Using own data with included `Dataset`s
+
+Gluon has a number of different [`Dataset`](https://mxnet.incubator.apache.org/api/python/gluon/data.html?highlight=dataset#mxnet.gluon.data.Dataset) classes for working with your own image data straight out-of-the-box. You can get started quickly using the [`mxnet.gluon.data.vision.datasets.ImageFolderDataset`](https://mxnet.incubator.apache.org/api/python/gluon/data.html?highlight=imagefolderdataset#mxnet.gluon.data.vision.datasets.ImageFolderDataset) which loads images directly from a user-defined folder, and infers the label (i.e. class) from the folders.
+
+We will run through an example for image classification, but a similar process applies for other vision tasks. If you already have your own collection of images to work with you should partition your data into training and test sets, and place all objects of the same class into seperate folders. Similar to:
+```
+ ./images/train/car/abc.jpg
+ ./images/train/car/efg.jpg
+ ./images/train/bus/hij.jpg
+ ./images/train/bus/klm.jpg
+ ./images/test/car/xyz.jpg
+ ./images/test/bus/uvw.jpg
+```
+
+You can download the Caltech 101 dataset if you don't already have images to work with for this example, but please note the download is 126MB.
+
+```python
+
+data_folder = "data"
+dataset_name = "101_ObjectCategories"
+archive_file = "{}.tar.gz".format(dataset_name)
+archive_path = os.path.join(data_folder, archive_file)
+data_url = "https://s3.us-east-2.amazonaws.com/mxnet-public/"
+
+if not os.path.isfile(archive_path):
+ mx.test_utils.download("{}{}".format(data_url, archive_file), dirname = data_folder)
+ print('Extracting {} in {}...'.format(archive_file, data_folder))
+ tar = tarfile.open(archive_path, "r:gz")
+ tar.extractall(data_folder)
+ tar.close()
+ print('Data extracted.')
+```
+
+After downloading and extracting the data archive, we have two folders: `data/101_ObjectCategories` and `data/101_ObjectCategories_test`. We load the data into separate training and testing [`ImageFolderDataset`](https://mxnet.incubator.apache.org/api/python/gluon/data.html?highlight=imagefolderdataset#mxnet.gluon.data.vision.datasets.ImageFolderDataset)s.
+
+```python
+training_path = os.path.join(data_folder, dataset_name)
+testing_path = os.path.join(data_folder, "{}_test".format(dataset_name))
+```
+
+We instantiate the [`ImageFolderDataset`](https://mxnet.incubator.apache.org/api/python/gluon/data.html?highlight=imagefolderdataset#mxnet.gluon.data.vision.datasets.ImageFolderDataset)s by providing the path to the data, and the folder structure will be traversed to determine which image classes are available and which images correspond to each class. You must take care to ensure the same classes are both the training and testing datasets, otherwise the label encodings can get muddled.
+
+Optionally, you can pass a `transform` parameter to these [`Dataset`](https://mxnet.incubator.apache.org/api/python/gluon/data.html?highlight=dataset#mxnet.gluon.data.Dataset)s as we've seen before.
+
+
+```python
+train_dataset = mx.gluon.data.vision.datasets.ImageFolderDataset(training_path)
+test_dataset = mx.gluon.data.vision.datasets.ImageFolderDataset(testing_path)
+```
+
+Samples from these datasets are tuples of data and label. Images are loaded from disk, decoded and optionally transformed when the `__getitem__(i)` method is called (equivalent to `train_dataset[i]`).
+
+As with the Fashion MNIST dataset the labels will be integer encoded. You can use the `synsets` property of the [`ImageFolderDataset`](https://mxnet.incubator.apache.org/api/python/gluon/data.html?highlight=imagefolderdataset#mxnet.gluon.data.vision.datasets.ImageFolderDataset)s to retrieve the original descriptions (e.g. `train_dataset.synsets[i]`).
+
+
+```python
+sample_idx = 539
+sample = train_dataset[sample_idx]
+data = sample[0]
+label = sample[1]
+
+imshow(data.asnumpy(), cmap='gray')
+print("Data type: {}".format(data.dtype))
+print("Label: {}".format(label))
+print("Label description: {}".format(train_dataset.synsets[label]))
+assert label == 1
+```
+
+`Data type: `
+
+`Label: 1`
+
+`Label description: Faces_easy`
+
+
+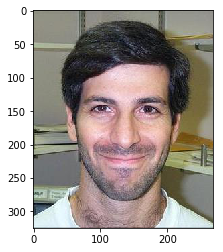
+
+
+# Using own data with custom `Dataset`s
+
+Sometimes you have data that doesn't quite fit the format expected by the included [`Dataset`](https://mxnet.incubator.apache.org/api/python/gluon/data.html?highlight=dataset#mxnet.gluon.data.Dataset)s. You might be able to preprocess your data to fit the expected format, but it is easy to create your own dataset to do this.
+
+All you need to do is create a class that implements a `__getitem__` method, that returns a sample (i.e. a tuple of [`mx.nd.NDArray`](https://mxnet.incubator.apache.org/api/python/ndarray/ndarray.html#mxnet.ndarray.NDArray)s).
+
+See the [Data Augmentation with Masks](http://mxnet.incubator.apache.org/tutorials/python/data_augmentation_with_masks.html) tutorial for an example of this.
+
+# Appendix: Upgrading from Module `DataIter` to Gluon `DataLoader`
+
+Before Gluon's [`DataLoader`](https://mxnet.incubator.apache.org/api/python/gluon/data.html?highlight=dataloader#mxnet.gluon.data.DataLoader), MXNet used [`DataIter`](https://mxnet.incubator.apache.org/api/python/io/io.html?highlight=dataiter#mxnet.io.DataIter) objects for loading data for training and testing. [`DataIter`](https://mxnet.incubator.apache.org/api/python/io/io.html?highlight=dataiter#mxnet.io.DataIter) has a similar interface for iterating through data, but it isn't directly compatible with typical Gluon [`DataLoader`](https://mxnet.incubator.apache.org/api/python/gluon/data.html?highlight=dataloader#mxnet.gluon.data.DataLoader) loops. Unlike Gluon [`DataLoader`](https://mxnet.incubator.apache.org/api/python/gluon/data.html?highlight=dataloader#mxnet.gluon.data.DataLoader) which often returns a tuple of `(data, label)`, a [`DataIter`](https://mxnet.incubator.apache.org/api/python/io/io.html?highlight=dataiter#mxnet.io.DataIter) returns a [`DataBatch`](https://mxnet.incubator.apache.org/api/python/io/io.html?highlight=databatch#mxnet.io.DataBatch) object that has `data` and `label` properties. Switching to [`DataLoader`](https://mxnet.incubator.apache.org/api/python/gluon/data.html?highlight=dataloader#mxnet.gluon.data.DataLoader)s is highly recommended when using Gluon, but you'll need to take care of pre-processing steps such as augmentations in a `transform` function.
+
+So you can get up and running with Gluon quicker if you have already imlemented complex pre-processing steps using [`DataIter`](https://mxnet.incubator.apache.org/api/python/io/io.html?highlight=dataiter#mxnet.io.DataIter), we have provided a simple class to wrap existing [`DataIter`](https://mxnet.incubator.apache.org/api/python/io/io.html?highlight=dataiter#mxnet.io.DataIter) objects so they can be used in a typical Gluon training loop. You can use this class for `DataIter`s such as [`mxnet.image.ImageIter`](https://mxnet.incubator.apache.org/api/python/image/image.html?highlight=imageiter#mxnet.image.ImageIter) and [`mxnet.io.ImageRecordIter`](https://mxnet.incubator.apache.org/api/python/io/io.html?highlight=imagere#mxnet.io.ImageRecordIter) that have single data and label arrays.
+
+
+```python
+class DataIterLoader():
+ def __init__(self, data_iter):
+ self.data_iter = data_iter
+
+ def __iter__(self):
+ self.data_iter.reset()
+ return self
+
+ def __next__(self):
+ batch = self.data_iter.__next__()
+ assert len(batch.data) == len(batch.label) == 1
+ data = batch.data[0]
+ label = batch.label[0]
+ return data, label
+
+ def next(self):
+ return self.__next__() # for Python 2
+```
+
+
+```python
+data_iter = mx.io.NDArrayIter(data=X, label=y, batch_size=5)
+data_iter_loader = DataIterLoader(data_iter)
+for X_batch, y_batch in data_iter_loader:
+ assert X_batch.shape == (5, 3)
+ assert y_batch.shape == (5, 1)
+```
+
diff --git a/docs/python_docs/python/tutorials/packages/gluon/data/index.rst b/docs/python_docs/python/tutorials/packages/gluon/data/index.rst
new file mode 100644
index 000000000000..9a1a0c263699
--- /dev/null
+++ b/docs/python_docs/python/tutorials/packages/gluon/data/index.rst
@@ -0,0 +1,40 @@
+.. Licensed to the Apache Software Foundation (ASF) under one
+ or more contributor license agreements. See the NOTICE file
+ distributed with this work for additional information
+ regarding copyright ownership. The ASF licenses this file
+ to you under the Apache License, Version 2.0 (the
+ "License"); you may not use this file except in compliance
+ with the License. You may obtain a copy of the License at
+
+ http://www.apache.org/licenses/LICENSE-2.0
+
+ Unless required by applicable law or agreed to in writing,
+ software distributed under the License is distributed on an
+ "AS IS" BASIS, WITHOUT WARRANTIES OR CONDITIONS OF ANY
+ KIND, either express or implied. See the License for the
+ specific language governing permissions and limitations
+ under the License.
+
+Data Tutorials
+===============
+
+These tutorials will help you learn how to load and use datasets with the Gluon API.
+
+ .. card::
+ :title: Data Augmentation
+ :link: data_augmentation.html
+
+ A guide to data augmentation.
+
+ .. card::
+ :title: Gluon Datasets and DataLoader
+ :link: datasets.html
+
+ A guide to loading data using the Gluon API.
+
+.. toctree::
+ :hidden:
+ :maxdepth: 1
+ :glob:
+
+ *
diff --git a/docs/python_docs/python/tutorials/packages/gluon/index.rst b/docs/python_docs/python/tutorials/packages/gluon/index.rst
index d97279465287..4435637ee381 100644
--- a/docs/python_docs/python/tutorials/packages/gluon/index.rst
+++ b/docs/python_docs/python/tutorials/packages/gluon/index.rst
@@ -70,15 +70,9 @@ Data
.. container:: cards
- .. card::
- :title: Data Loading
- :link: data/data.html
-
- How to load data for training.
-
.. card::
:title: Data Augmentation
- :link: data/data_augmentation.md
+ :link: data/data_augmentation.html
A guide to data augmentation.
diff --git a/docs/python_docs/python/tutorials/packages/index.rst b/docs/python_docs/python/tutorials/packages/index.rst
index 9fdd75c837f7..e43d658a0dbe 100644
--- a/docs/python_docs/python/tutorials/packages/index.rst
+++ b/docs/python_docs/python/tutorials/packages/index.rst
@@ -69,7 +69,7 @@ Shared APIs
.. card::
:title: Data APIs
- :link: data/index.html
+ :link: gluon/data/index.html
How to use MXNet's data APIs.
diff --git a/docs/python_docs/python/tutorials/performance/index.rst b/docs/python_docs/python/tutorials/performance/index.rst
index e547ecd297ed..b1f5c66c2001 100644
--- a/docs/python_docs/python/tutorials/performance/index.rst
+++ b/docs/python_docs/python/tutorials/performance/index.rst
@@ -111,7 +111,7 @@ Distributed Training
.. card::
:title: Data Parallelism in MXNet
- :link: /api/faq/multi_devices.html
+ :link: /api/faq/multi_device
An overview of distributed training strategies.
diff --git a/docs/static_site/src/_includes/get_started/macos/perl/perl.md b/docs/static_site/src/_includes/get_started/macos/perl/perl.md
index ab08489fdc56..45d59ddf78a6 100644
--- a/docs/static_site/src/_includes/get_started/macos/perl/perl.md
+++ b/docs/static_site/src/_includes/get_started/macos/perl/perl.md
@@ -1 +1 @@
-Refer to the [Perl section of installation guide](get_started/osx_setup.html#install-the-mxnet-package-for-perl).
\ No newline at end of file
+Refer to the [Perl section of installation guide](/get_started/osx_setup.html#install-the-mxnet-package-for-perl).
\ No newline at end of file
diff --git a/docs/static_site/src/_includes/get_started/macos/python/cpu/build-from-source.md b/docs/static_site/src/_includes/get_started/macos/python/cpu/build-from-source.md
index 01dd35b9f332..ee8e378ec20e 100644
--- a/docs/static_site/src/_includes/get_started/macos/python/cpu/build-from-source.md
+++ b/docs/static_site/src/_includes/get_started/macos/python/cpu/build-from-source.md
@@ -1,2 +1,2 @@
-To build from source, refer to the [MXNet macOS installation guide](get_started/osx_setup.html).
+To build from source, refer to the [MXNet macOS installation guide](/get_started/osx_setup.html).
MXNet developers should refer to the MXNet wiki's [Developer Setup on Mac](https://cwiki.apache.org/confluence/display/MXNET/MXNet+Developer+Setup+on+Mac).