diff --git a/contrib/SegmentAnything/LICENSE b/contrib/SegmentAnything/LICENSE
new file mode 100644
index 0000000000..261eeb9e9f
--- /dev/null
+++ b/contrib/SegmentAnything/LICENSE
@@ -0,0 +1,201 @@
+ Apache License
+ Version 2.0, January 2004
+ http://www.apache.org/licenses/
+
+ TERMS AND CONDITIONS FOR USE, REPRODUCTION, AND DISTRIBUTION
+
+ 1. Definitions.
+
+ "License" shall mean the terms and conditions for use, reproduction,
+ and distribution as defined by Sections 1 through 9 of this document.
+
+ "Licensor" shall mean the copyright owner or entity authorized by
+ the copyright owner that is granting the License.
+
+ "Legal Entity" shall mean the union of the acting entity and all
+ other entities that control, are controlled by, or are under common
+ control with that entity. For the purposes of this definition,
+ "control" means (i) the power, direct or indirect, to cause the
+ direction or management of such entity, whether by contract or
+ otherwise, or (ii) ownership of fifty percent (50%) or more of the
+ outstanding shares, or (iii) beneficial ownership of such entity.
+
+ "You" (or "Your") shall mean an individual or Legal Entity
+ exercising permissions granted by this License.
+
+ "Source" form shall mean the preferred form for making modifications,
+ including but not limited to software source code, documentation
+ source, and configuration files.
+
+ "Object" form shall mean any form resulting from mechanical
+ transformation or translation of a Source form, including but
+ not limited to compiled object code, generated documentation,
+ and conversions to other media types.
+
+ "Work" shall mean the work of authorship, whether in Source or
+ Object form, made available under the License, as indicated by a
+ copyright notice that is included in or attached to the work
+ (an example is provided in the Appendix below).
+
+ "Derivative Works" shall mean any work, whether in Source or Object
+ form, that is based on (or derived from) the Work and for which the
+ editorial revisions, annotations, elaborations, or other modifications
+ represent, as a whole, an original work of authorship. For the purposes
+ of this License, Derivative Works shall not include works that remain
+ separable from, or merely link (or bind by name) to the interfaces of,
+ the Work and Derivative Works thereof.
+
+ "Contribution" shall mean any work of authorship, including
+ the original version of the Work and any modifications or additions
+ to that Work or Derivative Works thereof, that is intentionally
+ submitted to Licensor for inclusion in the Work by the copyright owner
+ or by an individual or Legal Entity authorized to submit on behalf of
+ the copyright owner. For the purposes of this definition, "submitted"
+ means any form of electronic, verbal, or written communication sent
+ to the Licensor or its representatives, including but not limited to
+ communication on electronic mailing lists, source code control systems,
+ and issue tracking systems that are managed by, or on behalf of, the
+ Licensor for the purpose of discussing and improving the Work, but
+ excluding communication that is conspicuously marked or otherwise
+ designated in writing by the copyright owner as "Not a Contribution."
+
+ "Contributor" shall mean Licensor and any individual or Legal Entity
+ on behalf of whom a Contribution has been received by Licensor and
+ subsequently incorporated within the Work.
+
+ 2. Grant of Copyright License. Subject to the terms and conditions of
+ this License, each Contributor hereby grants to You a perpetual,
+ worldwide, non-exclusive, no-charge, royalty-free, irrevocable
+ copyright license to reproduce, prepare Derivative Works of,
+ publicly display, publicly perform, sublicense, and distribute the
+ Work and such Derivative Works in Source or Object form.
+
+ 3. Grant of Patent License. Subject to the terms and conditions of
+ this License, each Contributor hereby grants to You a perpetual,
+ worldwide, non-exclusive, no-charge, royalty-free, irrevocable
+ (except as stated in this section) patent license to make, have made,
+ use, offer to sell, sell, import, and otherwise transfer the Work,
+ where such license applies only to those patent claims licensable
+ by such Contributor that are necessarily infringed by their
+ Contribution(s) alone or by combination of their Contribution(s)
+ with the Work to which such Contribution(s) was submitted. If You
+ institute patent litigation against any entity (including a
+ cross-claim or counterclaim in a lawsuit) alleging that the Work
+ or a Contribution incorporated within the Work constitutes direct
+ or contributory patent infringement, then any patent licenses
+ granted to You under this License for that Work shall terminate
+ as of the date such litigation is filed.
+
+ 4. Redistribution. You may reproduce and distribute copies of the
+ Work or Derivative Works thereof in any medium, with or without
+ modifications, and in Source or Object form, provided that You
+ meet the following conditions:
+
+ (a) You must give any other recipients of the Work or
+ Derivative Works a copy of this License; and
+
+ (b) You must cause any modified files to carry prominent notices
+ stating that You changed the files; and
+
+ (c) You must retain, in the Source form of any Derivative Works
+ that You distribute, all copyright, patent, trademark, and
+ attribution notices from the Source form of the Work,
+ excluding those notices that do not pertain to any part of
+ the Derivative Works; and
+
+ (d) If the Work includes a "NOTICE" text file as part of its
+ distribution, then any Derivative Works that You distribute must
+ include a readable copy of the attribution notices contained
+ within such NOTICE file, excluding those notices that do not
+ pertain to any part of the Derivative Works, in at least one
+ of the following places: within a NOTICE text file distributed
+ as part of the Derivative Works; within the Source form or
+ documentation, if provided along with the Derivative Works; or,
+ within a display generated by the Derivative Works, if and
+ wherever such third-party notices normally appear. The contents
+ of the NOTICE file are for informational purposes only and
+ do not modify the License. You may add Your own attribution
+ notices within Derivative Works that You distribute, alongside
+ or as an addendum to the NOTICE text from the Work, provided
+ that such additional attribution notices cannot be construed
+ as modifying the License.
+
+ You may add Your own copyright statement to Your modifications and
+ may provide additional or different license terms and conditions
+ for use, reproduction, or distribution of Your modifications, or
+ for any such Derivative Works as a whole, provided Your use,
+ reproduction, and distribution of the Work otherwise complies with
+ the conditions stated in this License.
+
+ 5. Submission of Contributions. Unless You explicitly state otherwise,
+ any Contribution intentionally submitted for inclusion in the Work
+ by You to the Licensor shall be under the terms and conditions of
+ this License, without any additional terms or conditions.
+ Notwithstanding the above, nothing herein shall supersede or modify
+ the terms of any separate license agreement you may have executed
+ with Licensor regarding such Contributions.
+
+ 6. Trademarks. This License does not grant permission to use the trade
+ names, trademarks, service marks, or product names of the Licensor,
+ except as required for reasonable and customary use in describing the
+ origin of the Work and reproducing the content of the NOTICE file.
+
+ 7. Disclaimer of Warranty. Unless required by applicable law or
+ agreed to in writing, Licensor provides the Work (and each
+ Contributor provides its Contributions) on an "AS IS" BASIS,
+ WITHOUT WARRANTIES OR CONDITIONS OF ANY KIND, either express or
+ implied, including, without limitation, any warranties or conditions
+ of TITLE, NON-INFRINGEMENT, MERCHANTABILITY, or FITNESS FOR A
+ PARTICULAR PURPOSE. You are solely responsible for determining the
+ appropriateness of using or redistributing the Work and assume any
+ risks associated with Your exercise of permissions under this License.
+
+ 8. Limitation of Liability. In no event and under no legal theory,
+ whether in tort (including negligence), contract, or otherwise,
+ unless required by applicable law (such as deliberate and grossly
+ negligent acts) or agreed to in writing, shall any Contributor be
+ liable to You for damages, including any direct, indirect, special,
+ incidental, or consequential damages of any character arising as a
+ result of this License or out of the use or inability to use the
+ Work (including but not limited to damages for loss of goodwill,
+ work stoppage, computer failure or malfunction, or any and all
+ other commercial damages or losses), even if such Contributor
+ has been advised of the possibility of such damages.
+
+ 9. Accepting Warranty or Additional Liability. While redistributing
+ the Work or Derivative Works thereof, You may choose to offer,
+ and charge a fee for, acceptance of support, warranty, indemnity,
+ or other liability obligations and/or rights consistent with this
+ License. However, in accepting such obligations, You may act only
+ on Your own behalf and on Your sole responsibility, not on behalf
+ of any other Contributor, and only if You agree to indemnify,
+ defend, and hold each Contributor harmless for any liability
+ incurred by, or claims asserted against, such Contributor by reason
+ of your accepting any such warranty or additional liability.
+
+ END OF TERMS AND CONDITIONS
+
+ APPENDIX: How to apply the Apache License to your work.
+
+ To apply the Apache License to your work, attach the following
+ boilerplate notice, with the fields enclosed by brackets "[]"
+ replaced with your own identifying information. (Don't include
+ the brackets!) The text should be enclosed in the appropriate
+ comment syntax for the file format. We also recommend that a
+ file or class name and description of purpose be included on the
+ same "printed page" as the copyright notice for easier
+ identification within third-party archives.
+
+ Copyright [yyyy] [name of copyright owner]
+
+ Licensed under the Apache License, Version 2.0 (the "License");
+ you may not use this file except in compliance with the License.
+ You may obtain a copy of the License at
+
+ http://www.apache.org/licenses/LICENSE-2.0
+
+ Unless required by applicable law or agreed to in writing, software
+ distributed under the License is distributed on an "AS IS" BASIS,
+ WITHOUT WARRANTIES OR CONDITIONS OF ANY KIND, either express or implied.
+ See the License for the specific language governing permissions and
+ limitations under the License.
diff --git a/contrib/SegmentAnything/README.md b/contrib/SegmentAnything/README.md
new file mode 100644
index 0000000000..d692c05e5b
--- /dev/null
+++ b/contrib/SegmentAnything/README.md
@@ -0,0 +1,93 @@
+# Segment Anything with PaddleSeg
+
+## Reference
+
+> Alexander Kirillov, Eric Mintun, Nikhila Ravi, Hanzi Mao, Chloe Rolland, Laura Gustafson, Tete Xiao, Spencer Whitehead, Alexander C. Berg, Wan-Yen Lo, Piotr Dollár, Ross Girshick. [Segment Anything](https://ai.facebook.com/research/publications/segment-anything/).
+
+
+## Contents
+1. Overview
+2. Performance
+3. Try it by yourself with one line of code
+
+##
Overview
+
+We implemente the segment anything with the PaddlePaddle framework. **Segment Anything Model (SAM)** is a new task, model, and dataset for image segmentation. It can produce high quality object masks from different types of prompts including points, boxes, masks and text. Further, SAM can generate masks for all objects in whole image. It built a largest segmentation [dataset](https://segment-anything.com/dataset/index.html) to date (by far), with over 1 billion masks on 11M licensed and privacy respecting images. SAM has impressive zero-shot performance on a variety of tasks, even often competitive with or even superior to prior fully supervised results.
+
+We provide the pretrained model parameters of PaddlePaddle format, including [vit_b](https://bj.bcebos.com/paddleseg/dygraph/paddlesegAnything/vit_b/model.pdparams), [vit_l](https://bj.bcebos.com/paddleseg/dygraph/paddlesegAnything/vit_l/model.pdparams) and [vit_h](https://bj.bcebos.com/paddleseg/dygraph/paddlesegAnything/vit_h/model.pdparams).
+
+##
Performance
+
+
+

+
+
+
+##
Try it by yourself with one line of code
+
+### 1. Preparation
+* Install PaddlePaddle and relative environments based on the [installation guide](https://www.paddlepaddle.org.cn/en/install/quick?docurl=/documentation/docs/en/install/pip/linux-pip_en.html).
+* Install PaddleSeg based on the [reference](../../docs/install.md).
+* Clone the PaddleSeg reporitory:
+ ```bash
+ git clone https://github.com/PaddlePaddle/PaddleSeg.git
+ cd PaddleSeg
+ pip install -r requirements.txt
+ ```
+* Download the example image to ```contrib/SegmentAnything/examples```, and the file structure is as following:
+ ```bash
+ wget https://paddleseg.bj.bcebos.com/dygraph/demo/cityscapes_demo.png
+ ```
+
+ ```
+ PaddleSeg/contrib
+ ├── SegmentAnything
+ │ ├── examples
+ │ │ └── cityscapes_demo.png
+ │ ├── modeling
+ │ └── script
+
+ ```
+
+### 2. Segment the whole image on webpage.
+In this step, we start a gradio service with the following scrip on local machine and you can try out our project with your own images.
+
+1. Run the following script:
+ ```bash
+ python script/amg_paddle.py --model-type [vit_l/vit_b/vit_h] # default is vit_h
+
+ ```
+ Note:
+ * There are three model options for you, vit_b, vit_l and vit_h, represent vit_base, vit_large and vit_huge. Large model is more accurate and also slower. You can choose the model size based on your device.
+ * The test result shows that vit_h needs 16G video memory and needs around 10s to infer an image on V100.
+
+2. Open the webpage on your localhost: ```http://0.0.0.0:8017```
+
+3. Try it out by clear and upload the test image! Our example looks like:
+
+
+
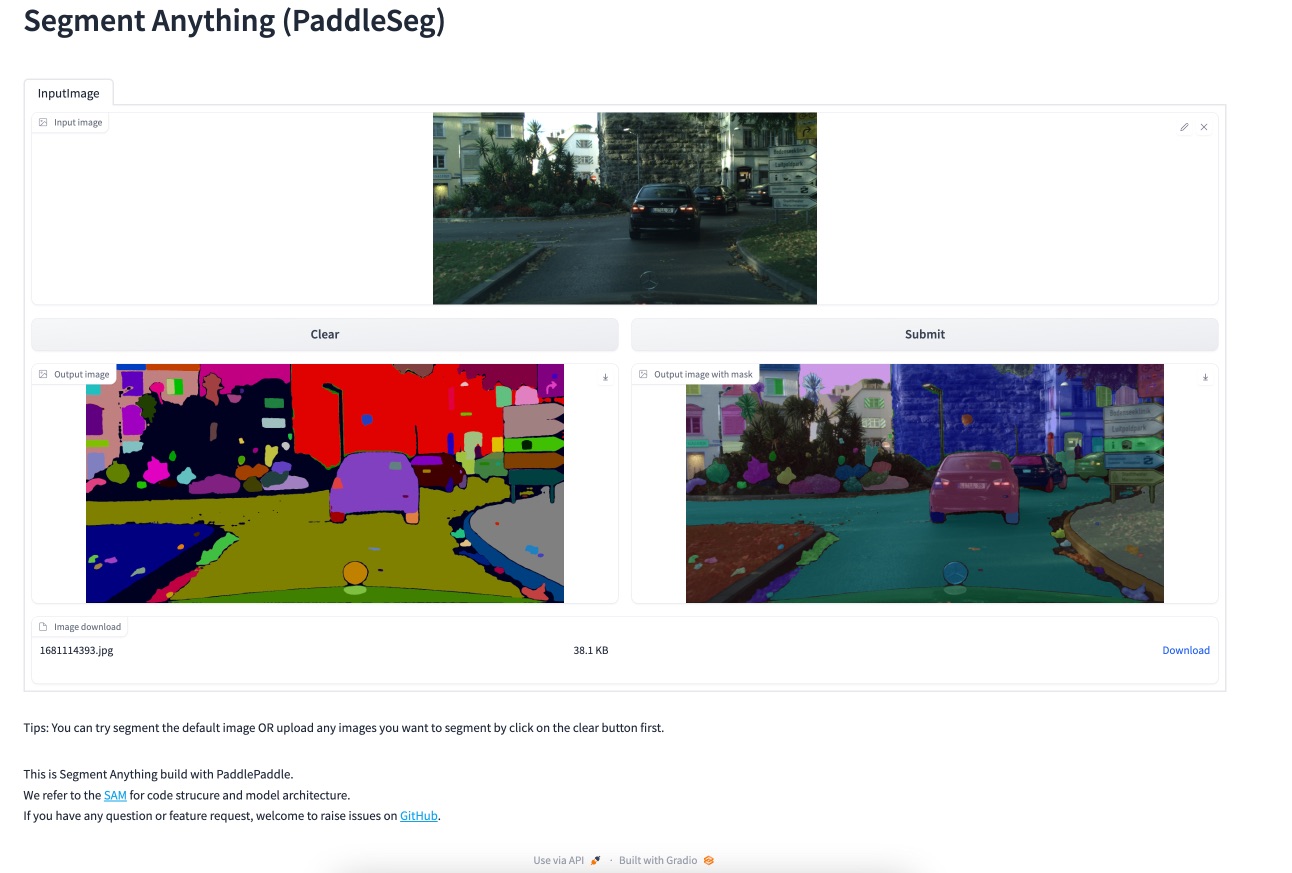
+
+
+### 3. Segment the object with prompts
+You can run the following commands to produce masks from different types of prompts including points, boxes, and masks, as follow:
+
+
+1. Box prompt
+
+```bash
+python script/promt_predict.py --input_path xxx.png --box_prompt 1050 370 1500 700 --model-type [vit_l/vit_b/vit_h] # default is vit_h
+```
+
+2. Point prompt
+```bash
+python script/promt_predict.py --input_path xxx.png --point_prompt 1200 450 --model-type [vit_l/vit_b/vit_h] # default is vit_h
+```
+
+3. Mask prompt
+```bash
+python script/promt_predict.py --input_path xxx.png --mask_prompt xxx.png --model-type [vit_l/vit_b/vit_h] # default is vit_h
+```
+
+Note:
+* mask_prompt is the path of a binary image.
diff --git a/contrib/SegmentAnything/scripts/amg_paddle.py b/contrib/SegmentAnything/scripts/amg_paddle.py
new file mode 100644
index 0000000000..0819045c4f
--- /dev/null
+++ b/contrib/SegmentAnything/scripts/amg_paddle.py
@@ -0,0 +1,306 @@
+# Copyright (c) 2023 PaddlePaddle Authors. All Rights Reserved.
+#
+# Licensed under the Apache License, Version 2.0 (the "License");
+# you may not use this file except in compliance with the License.
+# You may obtain a copy of the License at
+#
+# http://www.apache.org/licenses/LICENSE-2.0
+#
+# Unless required by applicable law or agreed to in writing, software
+# distributed under the License is distributed on an "AS IS" BASIS,
+# WITHOUT WARRANTIES OR CONDITIONS OF ANY KIND, either express or implied.
+# See the License for the specific language governing permissions and
+# limitations under the License.
+
+# This implementation refers to: https://github.com/facebookresearch/segment-anything
+
+import os
+import sys
+sys.path.append(os.path.join(os.path.dirname(os.path.realpath(__file__)), ".."))
+
+import time
+import cv2 # type: ignore
+import argparse
+import numpy as np # type: ignore
+import paddle
+
+from segment_anything import SamAutomaticMaskGenerator, sam_model_registry
+from paddleseg.utils.visualize import get_pseudo_color_map, get_color_map_list
+
+ID_PHOTO_IMAGE_DEMO = "examples/cityscapes_demo.png"
+CACHE_DIR = ".temp"
+
+model_link = {
+ 'vit_h':
+ "https://bj.bcebos.com/paddleseg/dygraph/paddlesegAnything/vit_h/model.pdparams",
+ 'vit_l':
+ "https://bj.bcebos.com/paddleseg/dygraph/paddlesegAnything/vit_l/model.pdparams",
+ 'vit_b':
+ "https://bj.bcebos.com/paddleseg/dygraph/paddlesegAnything/vit_b/model.pdparams"
+}
+
+parser = argparse.ArgumentParser(description=(
+ "Runs automatic mask generation on an input image or directory of images, "
+ "and outputs masks as either PNGs or COCO-style RLEs. Requires open-cv, "
+ "as well as pycocotools if saving in RLE format."))
+
+parser.add_argument(
+ "--model-type",
+ type=str,
+ default="vit_l",
+ required=True,
+ help="The type of model to load, in ['vit_h', 'vit_l', 'vit_b']", )
+
+parser.add_argument(
+ "--convert-to-rle",
+ action="store_true",
+ help=(
+ "Save masks as COCO RLEs in a single json instead of as a folder of PNGs. "
+ "Requires pycocotools."), )
+
+amg_settings = parser.add_argument_group("AMG Settings")
+
+amg_settings.add_argument(
+ "--points-per-side",
+ type=int,
+ default=None,
+ help="Generate masks by sampling a grid over the image with this many points to a side.",
+)
+
+amg_settings.add_argument(
+ "--points-per-batch",
+ type=int,
+ default=None,
+ help="How many input points to process simultaneously in one batch.", )
+
+amg_settings.add_argument(
+ "--pred-iou-thresh",
+ type=float,
+ default=None,
+ help="Exclude masks with a predicted score from the model that is lower than this threshold.",
+)
+
+amg_settings.add_argument(
+ "--stability-score-thresh",
+ type=float,
+ default=None,
+ help="Exclude masks with a stability score lower than this threshold.", )
+
+amg_settings.add_argument(
+ "--stability-score-offset",
+ type=float,
+ default=None,
+ help="Larger values perturb the mask more when measuring stability score.",
+)
+
+amg_settings.add_argument(
+ "--box-nms-thresh",
+ type=float,
+ default=None,
+ help="The overlap threshold for excluding a duplicate mask.", )
+
+amg_settings.add_argument(
+ "--crop-n-layers",
+ type=int,
+ default=None,
+ help=(
+ "If >0, mask generation is run on smaller crops of the image to generate more masks. "
+ "The value sets how many different scales to crop at."), )
+
+amg_settings.add_argument(
+ "--crop-nms-thresh",
+ type=float,
+ default=None,
+ help="The overlap threshold for excluding duplicate masks across different crops.",
+)
+
+amg_settings.add_argument(
+ "--crop-overlap-ratio",
+ type=int,
+ default=None,
+ help="Larger numbers mean image crops will overlap more.", )
+
+amg_settings.add_argument(
+ "--crop-n-points-downscale-factor",
+ type=int,
+ default=None,
+ help="The number of points-per-side in each layer of crop is reduced by this factor.",
+)
+
+amg_settings.add_argument(
+ "--min-mask-region-area",
+ type=int,
+ default=None,
+ help=(
+ "Disconnected mask regions or holes with area smaller than this value "
+ "in pixels are removed by postprocessing."), )
+
+
+def get_amg_kwargs(args):
+ amg_kwargs = {
+ "points_per_side": args.points_per_side,
+ "points_per_batch": args.points_per_batch,
+ "pred_iou_thresh": args.pred_iou_thresh,
+ "stability_score_thresh": args.stability_score_thresh,
+ "stability_score_offset": args.stability_score_offset,
+ "box_nms_thresh": args.box_nms_thresh,
+ "crop_n_layers": args.crop_n_layers,
+ "crop_nms_thresh": args.crop_nms_thresh,
+ "crop_overlap_ratio": args.crop_overlap_ratio,
+ "crop_n_points_downscale_factor": args.crop_n_points_downscale_factor,
+ "min_mask_region_area": args.min_mask_region_area,
+ }
+ amg_kwargs = {k: v for k, v in amg_kwargs.items() if v is not None}
+ return amg_kwargs
+
+
+def delete_result():
+ """clear old result in `.temp`"""
+ results = sorted(os.listdir(CACHE_DIR))
+ for res in results:
+ if int(time.time()) - int(os.path.splitext(res)[0]) > 10000:
+ os.remove(os.path.join(CACHE_DIR, res))
+
+
+def download(img):
+ if not os.path.exists(CACHE_DIR):
+ os.makedirs(CACHE_DIR)
+ while True:
+ name = str(int(time.time()))
+ tmp_name = os.path.join(CACHE_DIR, name + '.jpg')
+ if not os.path.exists(tmp_name):
+ break
+ else:
+ time.sleep(1)
+
+ img.save(tmp_name, 'png')
+ return tmp_name
+
+
+def masks2pseudomap(masks):
+ result = np.ones(masks[0]["segmentation"].shape, dtype=np.uint8) * 255
+ for i, mask_data in enumerate(masks):
+ result[mask_data["segmentation"] == 1] = i + 1
+ pred_result = result
+ result = get_pseudo_color_map(result)
+
+ return pred_result, result
+
+
+def visualize(image, result, color_map, weight=0.6):
+ """
+ Convert predict result to color image, and save added image.
+
+ Args:
+ image (str): The path of origin image.
+ result (np.ndarray): The predict result of image.
+ color_map (list): The color used to save the prediction results.
+ save_dir (str): The directory for saving visual image. Default: None.
+ weight (float): The image weight of visual image, and the result weight is (1 - weight). Default: 0.6
+
+ Returns:
+ vis_result (np.ndarray): If `save_dir` is None, return the visualized result.
+ """
+
+ color_map = [color_map[i:i + 3] for i in range(0, len(color_map), 3)]
+ color_map = np.array(color_map).astype("uint8")
+ # Use OpenCV LUT for color mapping
+ c1 = cv2.LUT(result, color_map[:, 0])
+ c2 = cv2.LUT(result, color_map[:, 1])
+ c3 = cv2.LUT(result, color_map[:, 2])
+ pseudo_img = np.dstack((c3, c2, c1))
+
+ # im = cv2.imread(image)
+ vis_result = cv2.addWeighted(image, weight, pseudo_img, 1 - weight, 0)
+ return vis_result
+
+
+def gradio_display(generator):
+ import gradio as gr
+
+ def clear_image_all():
+ delete_result()
+ return None, None, None, None
+
+ def get_id_photo_output(img):
+ """
+ Get the special size and background photo.
+
+ Args:
+ img(numpy:ndarray): The image array.
+ size(str): The size user specified.
+ bg(str): The background color user specified.
+ download_size(str): The size for image saving.
+
+ """
+ predictor = generator
+ masks = predictor.generate(img)
+ pred_result, pseudo_map = masks2pseudomap(masks) # PIL Image
+ added_pseudo_map = visualize(
+ img, pred_result, color_map=get_color_map_list(256))
+ res_download = download(pseudo_map)
+
+ return pseudo_map, added_pseudo_map, res_download
+
+ with gr.Blocks() as demo:
+ gr.Markdown("""# Segment Anything (PaddleSeg) """)
+ with gr.Tab("InputImage"):
+ image_in = gr.Image(value=ID_PHOTO_IMAGE_DEMO, label="Input image")
+
+ with gr.Row():
+ image_clear_btn = gr.Button("Clear")
+ image_submit_btn = gr.Button("Submit")
+
+ with gr.Row():
+ img_out1 = gr.Image(
+ label="Output image", interactive=False).style(height=300)
+ img_out2 = gr.Image(
+ label="Output image with mask",
+ interactive=False).style(height=300)
+ downloaded_img = gr.File(label='Image download').style(height=50)
+
+ image_clear_btn.click(
+ fn=clear_image_all,
+ inputs=None,
+ outputs=[image_in, img_out1, img_out2, downloaded_img])
+
+ image_submit_btn.click(
+ fn=get_id_photo_output,
+ inputs=[image_in, ],
+ outputs=[img_out1, img_out2, downloaded_img])
+
+ gr.Markdown(
+ """Tips: You can try segment the default image OR upload any images you want to segment by click on the clear button first."""
+ )
+
+ gr.Markdown(
+ """This is Segment Anything build with PaddlePaddle.
+ We refer to the [SAM](https://github.com/facebookresearch/segment-anything) for code strucure and model architecture.
+ If you have any question or feature request, welcome to raise issues on [GitHub](https://github.com/PaddlePaddle/PaddleSeg/issues). """
+ )
+
+ gr.Button.style(1)
+
+ demo.launch(server_name="0.0.0.0", server_port=8017, share=True)
+
+
+def main(args: argparse.Namespace) -> None:
+ print("Loading model...")
+
+ sam = sam_model_registry[args.model_type](
+ checkpoint=model_link[args.model_type])
+ if paddle.is_compiled_with_cuda():
+ paddle.set_device("gpu")
+ else:
+ paddle.set_device("cpu")
+ output_mode = "coco_rle" if args.convert_to_rle else "binary_mask"
+ amg_kwargs = get_amg_kwargs(args)
+ generator = SamAutomaticMaskGenerator(
+ sam, output_mode=output_mode, **amg_kwargs)
+
+ gradio_display(generator)
+
+
+if __name__ == "__main__":
+ args = parser.parse_args()
+ main(args)
diff --git a/contrib/SegmentAnything/scripts/promt_predict.py b/contrib/SegmentAnything/scripts/promt_predict.py
new file mode 100644
index 0000000000..39f9b67bfd
--- /dev/null
+++ b/contrib/SegmentAnything/scripts/promt_predict.py
@@ -0,0 +1,131 @@
+# Copyright (c) 2023 PaddlePaddle Authors. All Rights Reserved.
+#
+# Licensed under the Apache License, Version 2.0 (the "License");
+# you may not use this file except in compliance with the License.
+# You may obtain a copy of the License at
+#
+# http://www.apache.org/licenses/LICENSE-2.0
+#
+# Unless required by applicable law or agreed to in writing, software
+# distributed under the License is distributed on an "AS IS" BASIS,
+# WITHOUT WARRANTIES OR CONDITIONS OF ANY KIND, either express or implied.
+# See the License for the specific language governing permissions and
+# limitations under the License.
+
+# This implementation refers to: https://github.com/facebookresearch/segment-anything
+
+import os
+import sys
+import argparse
+sys.path.append(os.path.join(os.path.dirname(os.path.realpath(__file__)), ".."))
+
+import paddle
+import cv2
+import numpy as np
+import matplotlib.pyplot as plt
+
+from paddleseg_anything.predictor import SamPredictor
+from paddleseg_anything.build_sam import sam_model_registry
+
+model_link = {
+ 'vit_h':
+ "https://bj.bcebos.com/paddleseg/dygraph/paddlesegAnything/vit_h/model.pdparams",
+ 'vit_l':
+ "https://bj.bcebos.com/paddleseg/dygraph/paddlesegAnything/vit_l/model.pdparams",
+ 'vit_b':
+ "https://bj.bcebos.com/paddleseg/dygraph/paddlesegAnything/vit_b/model.pdparams"
+}
+
+
+def get_args():
+ parser = argparse.ArgumentParser(
+ description='Segment image with point promp, box or mask')
+ # Parameters
+ parser.add_argument(
+ '--input_path', type=str, required=True, help='The directory of image.')
+ parser.add_argument(
+ "--model-type",
+ type=str,
+ default="vit_l",
+ required=True,
+ help="The type of model to load, in ['vit_h', 'vit_l', 'vit_b']", )
+ parser.add_argument(
+ '--point_prompt',
+ type=int,
+ nargs='+',
+ default=None,
+ help='point promt.')
+ parser.add_argument(
+ '--box_prompt',
+ type=int,
+ nargs='+',
+ default=None,
+ help='box promt format as xyxy.')
+ parser.add_argument(
+ '--mask_prompt', type=str, default=None, help='The path of mask.')
+ parser.add_argument(
+ '--output_path',
+ type=str,
+ default='./output/',
+ help='The directory for saving the results')
+ return parser.parse_args()
+
+
+def show_mask(mask, ax, random_color=False):
+ if random_color:
+ color = np.concatenate([np.random.random(3), np.array([0.6])], axis=0)
+ else:
+ color = np.array([30 / 255, 144 / 255, 255 / 255, 0.6])
+ h, w = mask.shape[-2:]
+ mask_image = mask.reshape(h, w, 1) * color.reshape(1, 1, -1)
+ ax.imshow(mask_image)
+
+
+def main(args):
+ if paddle.is_compiled_with_cuda():
+ paddle.set_device("gpu")
+ else:
+ paddle.set_device("cpu")
+ input_path = args.input_path
+ output_path = args.output_path
+ point, box, mask_path = args.point_prompt, args.box_prompt, args.mask_prompt
+ if point is not None:
+ point = np.array([point])
+ input_label = np.array([1])
+ else:
+ input_label = None
+ if box is not None:
+ box = np.array([[box[0], box[1]], [box[2], box[3]]])
+ if mask_path is not None:
+ mask = cv2.imread(mask_path, -1)
+ else:
+ mask = None
+
+ image = cv2.imread(input_path)
+ image = cv2.cvtColor(image, cv2.COLOR_BGR2RGB)
+ model = sam_model_registry[args.model_type](
+ checkpoint=model_link[args.model_type])
+ predictor = SamPredictor(model)
+ predictor.set_image(image)
+
+ masks, _, _ = predictor.predict(
+ point_coords=point,
+ point_labels=input_label,
+ box=box,
+ mask_input=mask,
+ multimask_output=True, )
+
+ plt.figure(figsize=(10, 10))
+ plt.imshow(image)
+ show_mask(masks[0], plt.gca())
+ plt.axis('off')
+ basename = os.path.basename(input_path)
+ if not os.path.exists(output_path):
+ os.makedirs(output_path)
+ path_output = os.path.join(output_path, basename)
+ plt.savefig(path_output)
+
+
+if __name__ == "__main__":
+ args = get_args()
+ main(args)
diff --git a/contrib/SegmentAnything/segment_anything/__init__.py b/contrib/SegmentAnything/segment_anything/__init__.py
new file mode 100644
index 0000000000..ed26fb8804
--- /dev/null
+++ b/contrib/SegmentAnything/segment_anything/__init__.py
@@ -0,0 +1,24 @@
+# Copyright (c) 2023 PaddlePaddle Authors. All Rights Reserved.
+#
+# Licensed under the Apache License, Version 2.0 (the "License");
+# you may not use this file except in compliance with the License.
+# You may obtain a copy of the License at
+#
+# http://www.apache.org/licenses/LICENSE-2.0
+#
+# Unless required by applicable law or agreed to in writing, software
+# distributed under the License is distributed on an "AS IS" BASIS,
+# WITHOUT WARRANTIES OR CONDITIONS OF ANY KIND, either express or implied.
+# See the License for the specific language governing permissions and
+# limitations under the License.
+
+# This implementation refers to: https://github.com/facebookresearch/segment-anything
+
+from .build_sam import (
+ build_sam,
+ build_sam_vit_h,
+ build_sam_vit_l,
+ build_sam_vit_b,
+ sam_model_registry, )
+from .predictor import SamPredictor
+from .automatic_mask_generator import SamAutomaticMaskGenerator
diff --git a/contrib/SegmentAnything/segment_anything/automatic_mask_generator.py b/contrib/SegmentAnything/segment_anything/automatic_mask_generator.py
new file mode 100644
index 0000000000..02dc4061b7
--- /dev/null
+++ b/contrib/SegmentAnything/segment_anything/automatic_mask_generator.py
@@ -0,0 +1,406 @@
+# Copyright (c) 2023 PaddlePaddle Authors. All Rights Reserved.
+#
+# Licensed under the Apache License, Version 2.0 (the "License");
+# you may not use this file except in compliance with the License.
+# You may obtain a copy of the License at
+#
+# http://www.apache.org/licenses/LICENSE-2.0
+#
+# Unless required by applicable law or agreed to in writing, software
+# distributed under the License is distributed on an "AS IS" BASIS,
+# WITHOUT WARRANTIES OR CONDITIONS OF ANY KIND, either express or implied.
+# See the License for the specific language governing permissions and
+# limitations under the License.
+
+# This implementation refers to: https://github.com/facebookresearch/segment-anything
+
+import numpy as np
+import paddle
+from paddle.vision.ops import nms
+
+from typing import Any, Dict, List, Optional, Tuple
+
+from .modeling import Sam
+from .predictor import SamPredictor
+from .utils.amg import (
+ MaskData,
+ area_from_rle,
+ batch_iterator,
+ batched_mask_to_box,
+ box_xyxy_to_xywh,
+ build_all_layer_point_grids,
+ calculate_stability_score,
+ coco_encode_rle,
+ generate_crop_boxes,
+ is_box_near_crop_edge,
+ mask_to_rle_paddle,
+ remove_small_regions,
+ rle_to_mask,
+ uncrop_boxes_xyxy,
+ uncrop_masks,
+ uncrop_points, )
+
+
+class SamAutomaticMaskGenerator:
+ def __init__(
+ self,
+ model: Sam,
+ points_per_side: Optional[int]=32,
+ points_per_batch: int=64,
+ pred_iou_thresh: float=0.88,
+ stability_score_thresh: float=0.95,
+ stability_score_offset: float=1.0,
+ box_nms_thresh: float=0.7,
+ crop_n_layers: int=0,
+ crop_nms_thresh: float=0.7,
+ crop_overlap_ratio: float=512 / 1500,
+ crop_n_points_downscale_factor: int=1,
+ point_grids: Optional[List[np.ndarray]]=None,
+ min_mask_region_area: int=0,
+ output_mode: str="binary_mask", ) -> None:
+ """
+ Using a SAM model, generates masks for the entire image.
+ Generates a grid of point prompts over the image, then filters
+ low quality and duplicate masks. The default settings are chosen
+ for SAM with a ViT-H backbone.
+
+ Arguments:
+ model (Sam): The SAM model to use for mask prediction.
+ points_per_side (int or None): The number of points to be sampled
+ along one side of the image. The total number of points is
+ points_per_side**2. If None, 'point_grids' must provide explicit
+ point sampling.
+ points_per_batch (int): Sets the number of points run simultaneously
+ by the model. Higher numbers may be faster but use more GPU memory.
+ pred_iou_thresh (float): A filtering threshold in [0,1], using the
+ model's predicted mask quality.
+ stability_score_thresh (float): A filtering threshold in [0,1], using
+ the stability of the mask under changes to the cutoff used to binarize
+ the model's mask predictions.
+ stability_score_offset (float): The amount to shift the cutoff when
+ calculated the stability score.
+ box_nms_thresh (float): The box IoU cutoff used by non-maximal
+ suppression to filter duplicate masks.
+ crops_n_layers (int): If >0, mask prediction will be run again on
+ crops of the image. Sets the number of layers to run, where each
+ layer has 2**i_layer number of image crops.
+ crops_nms_thresh (float): The box IoU cutoff used by non-maximal
+ suppression to filter duplicate masks between different crops.
+ crop_overlap_ratio (float): Sets the degree to which crops overlap.
+ In the first crop layer, crops will overlap by this fraction of
+ the image length. Later layers with more crops scale down this overlap.
+ crop_n_points_downscale_factor (int): The number of points-per-side
+ sampled in layer n is scaled down by crop_n_points_downscale_factor**n.
+ point_grids (list(np.ndarray) or None): A list over explicit grids
+ of points used for sampling, normalized to [0,1]. The nth grid in the
+ list is used in the nth crop layer. Exclusive with points_per_side.
+ min_mask_region_area (int): If >0, postprocessing will be applied
+ to remove disconnected regions and holes in masks with area smaller
+ than min_mask_region_area. Requires opencv.
+ output_mode (str): The form masks are returned in. Can be 'binary_mask',
+ 'uncompressed_rle', or 'coco_rle'. 'coco_rle' requires pycocotools.
+ For large resolutions, 'binary_mask' may consume large amounts of
+ memory.
+ """
+
+ assert (points_per_side is None) != (
+ point_grids is None
+ ), "Exactly one of points_per_side or point_grid must be provided."
+ if points_per_side is not None:
+ self.point_grids = build_all_layer_point_grids(
+ points_per_side,
+ crop_n_layers,
+ crop_n_points_downscale_factor, )
+ elif point_grids is not None:
+ self.point_grids = point_grids
+ else:
+ raise ValueError(
+ "Can't have both points_per_side and point_grid be None.")
+
+ assert output_mode in [
+ "binary_mask",
+ "uncompressed_rle",
+ "coco_rle",
+ ], f"Unknown output_mode {output_mode}."
+ if output_mode == "coco_rle":
+ from pycocotools import mask as mask_utils # type: ignore # noqa: F401
+
+ if min_mask_region_area > 0:
+ import cv2 # type: ignore # noqa: F401
+
+ self.predictor = SamPredictor(model)
+ self.points_per_batch = points_per_batch
+ self.pred_iou_thresh = pred_iou_thresh
+ self.stability_score_thresh = stability_score_thresh
+ self.stability_score_offset = stability_score_offset
+ self.box_nms_thresh = box_nms_thresh
+ self.crop_n_layers = crop_n_layers
+ self.crop_nms_thresh = crop_nms_thresh
+ self.crop_overlap_ratio = crop_overlap_ratio
+ self.crop_n_points_downscale_factor = crop_n_points_downscale_factor
+ self.min_mask_region_area = min_mask_region_area
+ self.output_mode = output_mode
+
+ @paddle.no_grad()
+ def generate(self, image: np.ndarray) -> List[Dict[str, Any]]:
+ """
+ Generates masks for the given image.
+
+ Arguments:
+ image (np.ndarray): The image to generate masks for, in HWC uint8 format.
+
+ Returns:
+ list(dict(str, any)): A list over records for masks. Each record is
+ a dict containing the following keys:
+ segmentation (dict(str, any) or np.ndarray): The mask. If
+ output_mode='binary_mask', is an array of shape HW. Otherwise,
+ is a dictionary containing the RLE.
+ bbox (list(float)): The box around the mask, in XYWH format.
+ area (int): The area in pixels of the mask.
+ predicted_iou (float): The model's own prediction of the mask's
+ quality. This is filtered by the pred_iou_thresh parameter.
+ point_coords (list(list(float))): The point coordinates input
+ to the model to generate this mask.
+ stability_score (float): A measure of the mask's quality. This
+ is filtered on using the stability_score_thresh parameter.
+ crop_box (list(float)): The crop of the image used to generate
+ the mask, given in XYWH format.
+ """
+ paddle.device.set_device('gpu')
+ # Generate masks
+ mask_data = self._generate_masks(image)
+
+ # Filter small disconnected regions and holes in masks
+ if self.min_mask_region_area > 0:
+ mask_data = self.postprocess_small_regions(
+ mask_data,
+ self.min_mask_region_area,
+ max(self.box_nms_thresh, self.crop_nms_thresh), )
+
+ # Encode masks
+ if self.output_mode == "coco_rle":
+ mask_data["segmentations"] = [
+ coco_encode_rle(rle) for rle in mask_data["rles"]
+ ]
+ elif self.output_mode == "binary_mask":
+ mask_data["segmentations"] = [
+ rle_to_mask(rle) for rle in mask_data["rles"]
+ ]
+ else:
+ mask_data["segmentations"] = mask_data["rles"]
+
+ # Write mask records
+ curr_anns = []
+ for idx in range(len(mask_data["segmentations"])):
+ ann = {
+ "segmentation": mask_data["segmentations"][idx],
+ "area": area_from_rle(mask_data["rles"][idx]),
+ "bbox": box_xyxy_to_xywh(mask_data["boxes"][idx]).tolist(),
+ "predicted_iou": mask_data["iou_preds"][idx].item(), #.item(),
+ "point_coords": [mask_data["points"][idx].tolist()],
+ "stability_score": mask_data["stability_score"][idx].item(),
+ "crop_box":
+ box_xyxy_to_xywh(mask_data["crop_boxes"][idx]).tolist(),
+ }
+ curr_anns.append(ann)
+
+ return curr_anns
+
+ def _generate_masks(self, image: np.ndarray) -> MaskData:
+ def box_area(boxes):
+ """
+ Computes the area of a set of bounding boxes, which are specified by their
+ (x1, y1, x2, y2) coordinates.
+
+ Args:
+ boxes (Tensor[N, 4]): boxes for which the area will be computed. They
+ are expected to be in (x1, y1, x2, y2) format with
+ ``0 <= x1 < x2`` and ``0 <= y1 < y2``.
+
+ Returns:
+ Tensor[N]: the area for each box
+ """
+ if "float" in boxes.dtype:
+ boxes = boxes.cast('float64')
+ elif "int" in boxes.dtype:
+ boxes = boxes.cast('int64')
+
+ boxes = boxes
+ return (boxes[:, 2] - boxes[:, 0]) * (boxes[:, 3] - boxes[:, 1])
+
+ orig_size = image.shape[:2]
+ crop_boxes, layer_idxs = generate_crop_boxes(
+ orig_size, self.crop_n_layers, self.crop_overlap_ratio)
+
+ # Iterate over image crops
+ data = MaskData()
+ for crop_box, layer_idx in zip(crop_boxes, layer_idxs):
+ crop_data = self._process_crop(image, crop_box, layer_idx,
+ orig_size)
+ data.cat(crop_data)
+
+ # Remove duplicate masks between crops
+ if len(crop_boxes) > 1:
+ # Prefer masks from smaller crops
+ scores = 1 / box_area(data["crop_boxes"])
+ scores = scores.to(data["boxes"].device)
+ keep_by_nms = nms(
+ data["boxes"].cast('float32'),
+ scores=scores,
+ category_idxs=paddle.zeros(len(data["boxes"])), # categories
+ iou_threshold=self.crop_nms_thresh, )
+ data.filter(keep_by_nms)
+
+ data.to_numpy()
+ return data
+
+ def _process_crop(
+ self,
+ image: np.ndarray,
+ crop_box: List[int],
+ crop_layer_idx: int,
+ orig_size: Tuple[int, ...], ) -> MaskData:
+ # Crop the image and calculate embeddings
+ x0, y0, x1, y1 = crop_box
+ cropped_im = image[y0:y1, x0:x1, :]
+ cropped_im_size = cropped_im.shape[:2]
+ self.predictor.set_image(cropped_im)
+
+ # Get points for this crop
+ points_scale = np.array(cropped_im_size)[None, ::-1]
+ points_for_image = self.point_grids[crop_layer_idx] * points_scale
+
+ # Generate masks for this crop in batches
+ data = MaskData()
+ for (points, ) in batch_iterator(self.points_per_batch,
+ points_for_image):
+ batch_data = self._process_batch(points, cropped_im_size, crop_box,
+ orig_size)
+ data.cat(batch_data)
+ del batch_data
+ self.predictor.reset_image()
+
+ # Remove duplicates within this crop.
+ keep_by_nms = nms(
+ data["boxes"].cast('float32'),
+ scores=data["iou_preds"],
+ #category_idxs=paddle.zeros([len(data["boxes"])]), # categories
+ iou_threshold=self.box_nms_thresh, )
+ data.filter(keep_by_nms)
+
+ # Return to the original image frame
+ data["boxes"] = uncrop_boxes_xyxy(data["boxes"], crop_box)
+ data["points"] = uncrop_points(data["points"], crop_box)
+ data["crop_boxes"] = paddle.to_tensor(
+ [crop_box for _ in range(len(data["rles"]))])
+ return data
+
+ def _process_batch(
+ self,
+ points: np.ndarray,
+ im_size: Tuple[int, ...],
+ crop_box: List[int],
+ orig_size: Tuple[int, ...], ) -> MaskData:
+ orig_h, orig_w = orig_size
+
+ # Run model on this batch
+ transformed_points = self.predictor.transform.apply_coords(points,
+ im_size)
+ in_points = paddle.to_tensor(transformed_points)
+ in_labels = paddle.ones(shape=[in_points.shape[0], ], dtype='int64')
+ masks, iou_preds, _ = self.predictor.predict_paddle(
+ in_points[:, None, :],
+ in_labels[:, None],
+ multimask_output=True,
+ return_logits=True, )
+
+ # Serialize predictions and store in MaskData
+ data = MaskData(
+ masks=masks.flatten(0, 1),
+ iou_preds=iou_preds.flatten(0, 1),
+ points=paddle.to_tensor(points.repeat(
+ masks.shape[1], axis=0)), )
+ del masks
+
+ # Filter by predicted IoU
+ if self.pred_iou_thresh > 0.0:
+ keep_mask = data["iou_preds"] > self.pred_iou_thresh
+ data.filter(keep_mask)
+
+ # Calculate stability score
+ data["stability_score"] = calculate_stability_score(
+ data["masks"], self.predictor.model.mask_threshold,
+ self.stability_score_offset)
+
+ if self.stability_score_thresh > 0.0:
+ keep_mask = data["stability_score"] >= self.stability_score_thresh
+ data.filter(keep_mask)
+
+ # Threshold masks and calculate boxes
+ data["masks"] = (data["masks"] > self.predictor.model.mask_threshold
+ ).cast(paddle.float32)
+ data["boxes"] = batched_mask_to_box(data["masks"])
+
+ # Filter boxes that touch crop boundaries
+ keep_mask = ~is_box_near_crop_edge(data["boxes"], crop_box,
+ [0, 0, orig_w, orig_h])
+ if not paddle.all(keep_mask):
+ data.filter(keep_mask)
+
+ # Compress to RLE
+ data["masks"] = uncrop_masks(data["masks"], crop_box, orig_h, orig_w)
+ data["rles"] = mask_to_rle_paddle(data["masks"].cast(paddle.float32))
+ del data["masks"]
+
+ return data
+
+ @staticmethod
+ def postprocess_small_regions(mask_data: MaskData,
+ min_area: int,
+ nms_thresh: float) -> MaskData:
+ """
+ Removes small disconnected regions and holes in masks, then reruns
+ box NMS to remove any new duplicates.
+
+ Edits mask_data in place.
+
+ Requires open-cv as a dependency.
+ """
+ if len(mask_data["rles"]) == 0:
+ return mask_data
+
+ # Filter small disconnected regions and holes
+ new_masks = []
+ scores = []
+ for rle in mask_data["rles"]:
+ mask = rle_to_mask(rle)
+
+ mask, changed = remove_small_regions(mask, min_area, mode="holes")
+ unchanged = not changed
+ mask, changed = remove_small_regions(mask, min_area, mode="islands")
+ unchanged = unchanged and not changed
+
+ new_masks.append(paddle.to_tensor(mask).unsqueeze(0))
+ # Give score=0 to changed masks and score=1 to unchanged masks
+ # so NMS will prefer ones that didn't need postprocessing
+ scores.append(float(unchanged))
+
+ # Recalculate boxes and remove any new duplicates
+ masks = paddle.concat(new_masks, axis=0)
+ boxes = batched_mask_to_box(masks)
+ keep_by_nms = nms(
+ boxes.cast('float32'),
+ scores=paddle.to_tensor(scores),
+ category_idxs=paddle.zeros(len(boxes)), # categories
+ iou_threshold=nms_thresh, )
+
+ # Only recalculate RLEs for masks that have changed
+ for i_mask in keep_by_nms:
+ if scores[i_mask] == 0.0:
+ mask_paddle = masks[i_mask].unsqueeze(0)
+ mask_data["rles"][i_mask] = mask_to_rle_paddle(mask_paddle)[0]
+ mask_data["boxes"][i_mask] = boxes[
+ i_mask] # update res directly
+ mask_data.filter(keep_by_nms)
+
+ return mask_data
diff --git a/contrib/SegmentAnything/segment_anything/build_sam.py b/contrib/SegmentAnything/segment_anything/build_sam.py
new file mode 100644
index 0000000000..f19d033eb8
--- /dev/null
+++ b/contrib/SegmentAnything/segment_anything/build_sam.py
@@ -0,0 +1,108 @@
+# Copyright (c) 2023 PaddlePaddle Authors. All Rights Reserved.
+#
+# Licensed under the Apache License, Version 2.0 (the "License");
+# you may not use this file except in compliance with the License.
+# You may obtain a copy of the License at
+#
+# http://www.apache.org/licenses/LICENSE-2.0
+#
+# Unless required by applicable law or agreed to in writing, software
+# distributed under the License is distributed on an "AS IS" BASIS,
+# WITHOUT WARRANTIES OR CONDITIONS OF ANY KIND, either express or implied.
+# See the License for the specific language governing permissions and
+# limitations under the License.
+
+# This implementation refers to: https://github.com/facebookresearch/segment-anything
+import paddle
+
+from functools import partial
+
+from paddleseg import utils
+
+from .modeling import ImageEncoderViT, MaskDecoder, PromptEncoder, Sam, TwoWayTransformer
+
+
+def build_sam_vit_h(checkpoint=None):
+ return _build_sam(
+ encoder_embed_dim=1280,
+ encoder_depth=32,
+ encoder_num_heads=16,
+ encoder_global_attn_indexes=[7, 15, 23, 31],
+ checkpoint=checkpoint, )
+
+
+build_sam = build_sam_vit_h
+
+
+def build_sam_vit_l(checkpoint=None):
+ return _build_sam(
+ encoder_embed_dim=1024,
+ encoder_depth=24,
+ encoder_num_heads=16,
+ encoder_global_attn_indexes=[5, 11, 17, 23],
+ checkpoint=checkpoint, )
+
+
+def build_sam_vit_b(checkpoint=None):
+ return _build_sam(
+ encoder_embed_dim=768,
+ encoder_depth=12,
+ encoder_num_heads=12,
+ encoder_global_attn_indexes=[2, 5, 8, 11],
+ checkpoint=checkpoint, )
+
+
+sam_model_registry = {
+ "default": build_sam,
+ "vit_h": build_sam,
+ "vit_l": build_sam_vit_l,
+ "vit_b": build_sam_vit_b,
+}
+
+
+def _build_sam(
+ encoder_embed_dim,
+ encoder_depth,
+ encoder_num_heads,
+ encoder_global_attn_indexes,
+ checkpoint=None, ):
+ prompt_embed_dim = 256
+ image_size = 1024
+ vit_patch_size = 16
+ image_embedding_size = image_size // vit_patch_size
+ sam = Sam(
+ image_encoder=ImageEncoderViT(
+ depth=encoder_depth,
+ embed_dim=encoder_embed_dim,
+ img_size=image_size,
+ mlp_ratio=4,
+ norm_layer=partial(
+ paddle.nn.LayerNorm, epsilon=1e-6),
+ num_heads=encoder_num_heads,
+ patch_size=vit_patch_size,
+ qkv_bias=True,
+ use_rel_pos=True,
+ global_attn_indexes=encoder_global_attn_indexes,
+ window_size=14,
+ out_chans=prompt_embed_dim, ),
+ prompt_encoder=PromptEncoder(
+ embed_dim=prompt_embed_dim,
+ image_embedding_size=(image_embedding_size, image_embedding_size),
+ input_image_size=(image_size, image_size),
+ mask_in_chans=16, ),
+ mask_decoder=MaskDecoder(
+ num_multimask_outputs=3,
+ transformer=TwoWayTransformer(
+ depth=2,
+ embedding_dim=prompt_embed_dim,
+ mlp_dim=2048,
+ num_heads=8, ),
+ transformer_dim=prompt_embed_dim,
+ iou_head_depth=3,
+ iou_head_hidden_dim=256, ),
+ pixel_mean=[123.675, 116.28, 103.53],
+ pixel_std=[58.395, 57.12, 57.375], )
+ sam.eval()
+ if checkpoint is not None:
+ utils.load_entire_model(sam, checkpoint)
+ return sam
diff --git a/contrib/SegmentAnything/segment_anything/modeling/__init__.py b/contrib/SegmentAnything/segment_anything/modeling/__init__.py
new file mode 100644
index 0000000000..fe9172c737
--- /dev/null
+++ b/contrib/SegmentAnything/segment_anything/modeling/__init__.py
@@ -0,0 +1,21 @@
+# Copyright (c) 2023 PaddlePaddle Authors. All Rights Reserved.
+#
+# Licensed under the Apache License, Version 2.0 (the "License");
+# you may not use this file except in compliance with the License.
+# You may obtain a copy of the License at
+#
+# http://www.apache.org/licenses/LICENSE-2.0
+#
+# Unless required by applicable law or agreed to in writing, software
+# distributed under the License is distributed on an "AS IS" BASIS,
+# WITHOUT WARRANTIES OR CONDITIONS OF ANY KIND, either express or implied.
+# See the License for the specific language governing permissions and
+# limitations under the License.
+
+# This implementation refers to: https://github.com/facebookresearch/segment-anything
+
+from .sam import Sam
+from .image_encoder import ImageEncoderViT
+from .mask_decoder import MaskDecoder
+from .prompt_encoder import PromptEncoder
+from .transformer import TwoWayTransformer
diff --git a/contrib/SegmentAnything/segment_anything/modeling/common.py b/contrib/SegmentAnything/segment_anything/modeling/common.py
new file mode 100644
index 0000000000..cebf1c107a
--- /dev/null
+++ b/contrib/SegmentAnything/segment_anything/modeling/common.py
@@ -0,0 +1,54 @@
+# Copyright (c) 2023 PaddlePaddle Authors. All Rights Reserved.
+#
+# Licensed under the Apache License, Version 2.0 (the "License");
+# you may not use this file except in compliance with the License.
+# You may obtain a copy of the License at
+#
+# http://www.apache.org/licenses/LICENSE-2.0
+#
+# Unless required by applicable law or agreed to in writing, software
+# distributed under the License is distributed on an "AS IS" BASIS,
+# WITHOUT WARRANTIES OR CONDITIONS OF ANY KIND, either express or implied.
+# See the License for the specific language governing permissions and
+# limitations under the License.
+
+# This implementation refers to: https://github.com/facebookresearch/segment-anything
+
+import paddle
+import paddle.nn as nn
+from typing import Type
+
+
+class MLPBlock(nn.Layer):
+ def __init__(self,
+ embedding_dim: int,
+ mlp_dim: int,
+ act: Type[nn.Layer]=nn.GELU) -> None:
+ super().__init__()
+ self.lin1 = nn.Linear(embedding_dim, mlp_dim)
+ self.lin2 = nn.Linear(mlp_dim, embedding_dim)
+ self.act = act()
+
+ def forward(self, x: paddle.Tensor) -> paddle.Tensor:
+ return self.lin2(self.act(self.lin1(x)))
+
+
+class LayerNorm2d(nn.Layer):
+ def __init__(self, num_channels: int, eps: float=1e-06) -> None:
+ super().__init__()
+ self.weight = paddle.create_parameter(
+ shape=[num_channels],
+ dtype='float32',
+ default_initializer=nn.initializer.Constant(value=1.0))
+ self.bias = paddle.create_parameter(
+ shape=[num_channels],
+ dtype='float32',
+ default_initializer=nn.initializer.Constant(value=0.0))
+ self.eps = eps
+
+ def forward(self, x: paddle.Tensor) -> paddle.Tensor:
+ u = x.mean(1, keepdim=True)
+ s = (x - u).pow(2).mean(1, keepdim=True)
+ x = (x - u) / paddle.sqrt(s + self.eps)
+ x = self.weight[:, (None), (None)] * x + self.bias[:, (None), (None)]
+ return x
diff --git a/contrib/SegmentAnything/segment_anything/modeling/image_encoder.py b/contrib/SegmentAnything/segment_anything/modeling/image_encoder.py
new file mode 100644
index 0000000000..f52a22b6d2
--- /dev/null
+++ b/contrib/SegmentAnything/segment_anything/modeling/image_encoder.py
@@ -0,0 +1,492 @@
+# Copyright (c) 2023 PaddlePaddle Authors. All Rights Reserved.
+#
+# Licensed under the Apache License, Version 2.0 (the "License");
+# you may not use this file except in compliance with the License.
+# You may obtain a copy of the License at
+#
+# http://www.apache.org/licenses/LICENSE-2.0
+#
+# Unless required by applicable law or agreed to in writing, software
+# distributed under the License is distributed on an "AS IS" BASIS,
+# WITHOUT WARRANTIES OR CONDITIONS OF ANY KIND, either express or implied.
+# See the License for the specific language governing permissions and
+# limitations under the License.
+
+# This implementation refers to: https://github.com/facebookresearch/segment-anything
+
+import sys
+import os
+sys.path.append(os.path.join(os.path.dirname(os.path.realpath(__file__)), "."))
+
+from functools import partial
+from typing import Optional, Tuple, Type
+
+import paddle
+import paddle.nn as nn
+import paddle.nn.functional as F
+
+from .common import LayerNorm2d, MLPBlock
+
+
+# This class and its supporting functions below lightly adapted from the ViTDet backbone available at: https://github.com/facebookresearch/detectron2/blob/main/detectron2/modeling/backbone/vit.py # noqa
+class ImageEncoderViT(nn.Layer):
+ def __init__(
+ self,
+ img_size: int=1024,
+ patch_size: int=16,
+ in_chans: int=3,
+ embed_dim: int=768,
+ depth: int=12,
+ num_heads: int=12,
+ mlp_ratio: float=4.0,
+ out_chans: int=256,
+ qkv_bias: bool=True,
+ norm_layer: Type[nn.Layer]=nn.LayerNorm,
+ act_layer: Type[nn.Layer]=nn.GELU,
+ use_abs_pos: bool=True,
+ use_rel_pos: bool=False,
+ rel_pos_zero_init: bool=True,
+ window_size: int=0,
+ global_attn_indexes: Tuple[int, ...]=(), ) -> None:
+ """
+ Args:
+ img_size (int): Input image size.
+ patch_size (int): Patch size.
+ in_chans (int): Number of input image channels.
+ embed_dim (int): Patch embedding dimension.
+ depth (int): Depth of ViT.
+ num_heads (int): Number of attention heads in each ViT block.
+ mlp_ratio (float): Ratio of mlp hidden dim to embedding dim.
+ qkv_bias (bool): If True, add a learnable bias to query, key, value.
+ norm_layer (nn.Layer): Normalization layer.
+ act_layer (nn.Layer): Activation layer.
+ use_abs_pos (bool): If True, use absolute positional embeddings.
+ use_rel_pos (bool): If True, add relative positional embeddings to the attention map.
+ rel_pos_zero_init (bool): If True, zero initialize relative positional parameters.
+ window_size (int): Window size for window attention blocks.
+ global_attn_indexes (list): Indexes for blocks using global attention.
+ """
+ super().__init__()
+ self.img_size = img_size
+
+ self.patch_embed = PatchEmbed(
+ kernel_size=(patch_size, patch_size),
+ stride=(patch_size, patch_size),
+ in_chans=in_chans,
+ embed_dim=embed_dim, )
+
+ self.pos_embed = None
+ if use_abs_pos:
+ # Initialize absolute positional embedding with pretrain image size.
+ self.pos_embed = paddle.create_parameter(
+ shape=[
+ 1, img_size // patch_size, img_size // patch_size, embed_dim
+ ],
+ dtype='float32',
+ default_initializer=nn.initializer.Constant(value=0.0))
+ # self.pos_embed = nn.Parameter(paddle.zeros(1, img_size // patch_size, img_size // patch_size, embed_dim))
+
+ self.blocks = nn.LayerList()
+ for i in range(depth):
+ block = Block(
+ dim=embed_dim,
+ num_heads=num_heads,
+ mlp_ratio=mlp_ratio,
+ qkv_bias=qkv_bias,
+ norm_layer=norm_layer,
+ act_layer=act_layer,
+ use_rel_pos=use_rel_pos,
+ rel_pos_zero_init=rel_pos_zero_init,
+ window_size=window_size if i not in global_attn_indexes else 0,
+ input_size=(img_size // patch_size, img_size // patch_size), )
+ self.blocks.append(block)
+
+ self.neck = nn.Sequential(
+ nn.Conv2D(
+ embed_dim,
+ out_chans,
+ kernel_size=1,
+ bias_attr=False, ),
+ LayerNorm2d(out_chans),
+ nn.Conv2D(
+ out_chans,
+ out_chans,
+ kernel_size=3,
+ padding=1,
+ bias_attr=False, ),
+ LayerNorm2d(out_chans), )
+
+ def forward(self, x: paddle.Tensor) -> paddle.Tensor:
+ x = self.patch_embed(x)
+ if self.pos_embed is not None:
+ x = x + self.pos_embed
+
+ for blk in self.blocks:
+ x = blk(x)
+
+ x = self.neck(x.transpose([0, 3, 1, 2]))
+
+ return x
+
+
+class Block(nn.Layer):
+ """Transformer blocks with support of window attention and residual propagation blocks"""
+
+ def __init__(
+ self,
+ dim: int,
+ num_heads: int,
+ mlp_ratio: float=4.0,
+ qkv_bias: bool=True,
+ norm_layer=nn.LayerNorm,
+ act_layer=nn.GELU,
+ use_rel_pos: bool=False,
+ rel_pos_zero_init: bool=True,
+ window_size: int=0,
+ input_size: Optional[Tuple[int, int]]=None, ) -> None:
+ """
+ Args:
+ dim (int): Number of input channels.
+ num_heads (int): Number of attention heads in each ViT block.
+ mlp_ratio (float): Ratio of mlp hidden dim to embedding dim.
+ qkv_bias (bool): If True, add a learnable bias to query, key, value.
+ norm_layer (nn.Layer): Normalization layer.
+ act_layer (nn.Layer): Activation layer.
+ use_rel_pos (bool): If True, add relative positional embeddings to the attention map.
+ rel_pos_zero_init (bool): If True, zero initialize relative positional parameters.
+ window_size (int): Window size for window attention blocks. If it equals 0, then
+ use global attention.
+ input_size (int or None): Input resolution for calculating the relative positional
+ parameter size.
+ """
+ super().__init__()
+ self.norm1 = norm_layer(dim)
+ self.attn = Attention(
+ dim,
+ num_heads=num_heads,
+ qkv_bias=qkv_bias,
+ use_rel_pos=use_rel_pos,
+ rel_pos_zero_init=rel_pos_zero_init,
+ input_size=input_size
+ if window_size == 0 else (window_size, window_size), )
+
+ self.norm2 = norm_layer(dim)
+ self.mlp = MLPBlock(
+ embedding_dim=dim, mlp_dim=int(dim * mlp_ratio), act=act_layer)
+
+ self.window_size = window_size
+
+ def forward(self, x: paddle.Tensor) -> paddle.Tensor:
+ shortcut = x
+ x = self.norm1(x)
+ # Window partition
+ if self.window_size > 0:
+ H, W = x.shape[1], x.shape[2]
+ x, pad_hw = window_partition(x, self.window_size)
+
+ x = self.attn(x)
+ # Reverse window partition
+ if self.window_size > 0:
+ x = window_unpartition(x, self.window_size, pad_hw, (H, W))
+
+ x = shortcut + x
+ x = x + self.mlp(self.norm2(x))
+
+ return x
+
+
+class Attention(nn.Layer):
+ """Multi-head Attention block with relative position embeddings."""
+
+ def __init__(
+ self,
+ dim: int,
+ num_heads: int=8,
+ qkv_bias: bool=True,
+ use_rel_pos: bool=False,
+ rel_pos_zero_init: bool=True,
+ input_size: Optional[Tuple[int, int]]=None, ) -> None:
+ """
+ Args:
+ dim (int): Number of input channels.
+ num_heads (int): Number of attention heads.
+ qkv_bias (bool: If True, add a learnable bias to query, key, value.
+ rel_pos (bool): If True, add relative positional embeddings to the attention map.
+ rel_pos_zero_init (bool): If True, zero initialize relative positional parameters.
+ input_size (int or None): Input resolution for calculating the relative positional
+ parameter size.
+ """
+ super().__init__()
+ self.num_heads = num_heads
+ head_dim = dim // num_heads
+ self.scale = head_dim**-0.5
+
+ self.qkv = nn.Linear(
+ dim, dim * 3, bias_attr=qkv_bias if not qkv_bias else None)
+ self.proj = nn.Linear(dim, dim)
+
+ self.use_rel_pos = use_rel_pos
+ if self.use_rel_pos:
+ assert (
+ input_size is not None
+ ), "Input size must be provided if using relative positional encoding."
+ # initialize relative positional embeddings
+ self.rel_pos_h = paddle.create_parameter(
+ shape=[2 * input_size[0] - 1, head_dim],
+ dtype='float32',
+ default_initializer=nn.initializer.Constant(value=0.0))
+ self.rel_pos_w = paddle.create_parameter(
+ shape=[2 * input_size[1] - 1, head_dim],
+ dtype='float32',
+ default_initializer=nn.initializer.Constant(value=0.0))
+ # self.rel_pos_h = nn.Parameter(paddle.zeros(2 * input_size[0] - 1, head_dim))
+ # self.rel_pos_w = nn.Parameter(paddle.zeros(2 * input_size[1] - 1, head_dim))
+
+ def forward(self, x: paddle.Tensor) -> paddle.Tensor:
+ B, H, W, _ = x.shape
+ # qkv with shape (3, B, nHead, H * W, C)
+ qkv = self.qkv(x).reshape(
+ shape=[B, H * W, 3, self.num_heads, -1]).transpose([2, 0, 3, 1, 4])
+ # q, k, v with shape (B * nHead, H * W, C)
+
+ q, k, v = qkv.reshape(shape=[3, B * self.num_heads, H * W, -1]).unbind(
+ axis=0)
+
+ attn = (q * self.scale) @k.transpose([0, 2, 1])
+
+ if self.use_rel_pos:
+ attn = add_decomposed_rel_pos(attn, q, self.rel_pos_h,
+ self.rel_pos_w, (H, W), (H, W))
+
+ attn = F.softmax(attn, axis=-1)
+ x = (attn @v).reshape([B, self.num_heads, H, W, -1]).transpose(
+ [0, 2, 3, 1, 4]).reshape([B, H, W, -1])
+ x = self.proj(x)
+
+ return x
+
+
+def window_partition(x: paddle.Tensor,
+ window_size: int) -> Tuple[paddle.Tensor, Tuple[int, int]]:
+ """
+ Partition into non-overlapping windows with padding if needed.
+ Args:
+ x (tensor): input tokens with [B, H, W, C].
+ window_size (int): window size.
+
+ Returns:
+ windows: windows after partition with [B * num_windows, window_size, window_size, C].
+ (Hp, Wp): padded height and width before partition
+ """
+ B, H, W, C = x.shape
+
+ pad_h = (window_size - H % window_size) % window_size
+ pad_w = (window_size - W % window_size) % window_size
+ if pad_h > 0 or pad_w > 0:
+ x = paddle.nn.functional.pad(x=x, pad=(0, 0, 0, pad_w, 0, pad_h, 0,
+ 0)) # 每个维度分两位数进行pad
+ Hp, Wp = H + pad_h, W + pad_w
+
+ x = x.reshape(
+ [B, Hp // window_size, window_size, Wp // window_size, window_size, C])
+ windows = x.transpose([0, 1, 3, 2, 4, 5]).reshape(
+ [-1, window_size, window_size, C])
+ return windows, (Hp, Wp)
+
+
+def window_unpartition(windows: paddle.Tensor,
+ window_size: int,
+ pad_hw: Tuple[int, int],
+ hw: Tuple[int, int]) -> paddle.Tensor:
+ """
+ Window unpartition into original sequences and removing padding.
+ Args:
+ x (tensor): input tokens with [B * num_windows, window_size, window_size, C].
+ window_size (int): window size.
+ pad_hw (Tuple): padded height and width (Hp, Wp).
+ hw (Tuple): original height and width (H, W) before padding.
+
+ Returns:
+ x: unpartitioned sequences with [B, H, W, C].
+ """
+ Hp, Wp = pad_hw
+ H, W = hw
+ B = windows.shape[0] // (Hp * Wp // window_size // window_size)
+ x = windows.reshape([
+ B, Hp // window_size, Wp // window_size, window_size, window_size, -1
+ ])
+ x = x.transpose([0, 1, 3, 2, 4, 5]).reshape([B, Hp, Wp, -1])
+
+ if Hp > H or Wp > W:
+ x = x[:, :H, :W, :]
+ return x
+
+
+def get_rel_pos(q_size: int, k_size: int,
+ rel_pos: paddle.Tensor) -> paddle.Tensor:
+ """
+ Get relative positional embeddings according to the relative positions of
+ query and key sizes.
+ Args:
+ q_size (int): size of query q.
+ k_size (int): size of key k.
+ rel_pos (Tensor): relative position embeddings (L, C).
+
+ Returns:
+ Extracted positional embeddings according to relative positions.
+ """
+ max_rel_dist = int(2 * max(q_size, k_size) - 1)
+ # Interpolate rel pos if needed.
+ if rel_pos.shape[0] != max_rel_dist:
+ # Interpolate rel pos.
+ rel_pos_resized = F.interpolate(
+ rel_pos.reshape(1, rel_pos.shape[0], -1).transpose([0, 2, 1]),
+ size=max_rel_dist,
+ mode="linear", )
+ rel_pos_resized = rel_pos_resized.reshape([-1, max_rel_dist]).transpose(
+ [1, 0])
+ else:
+ rel_pos_resized = rel_pos
+
+ # Scale the coords with short length if shapes for q and k are different.
+ q_coords = paddle.arange(end=q_size)[:, None] * max(k_size / q_size, 1.0)
+ k_coords = paddle.arange(end=k_size)[None, :] * max(q_size / k_size, 1.0)
+ relative_coords = (q_coords - k_coords) + (k_size - 1) * max(q_size /
+ k_size, 1.0)
+
+ return rel_pos_resized[relative_coords.cast('int64')]
+
+
+def add_decomposed_rel_pos(
+ attn: paddle.Tensor,
+ q: paddle.Tensor,
+ rel_pos_h: paddle.Tensor,
+ rel_pos_w: paddle.Tensor,
+ q_size: Tuple[int, int],
+ k_size: Tuple[int, int], ) -> paddle.Tensor:
+ """
+ Calculate decomposed Relative Positional Embeddings from :paper:`mvitv2`.
+ https://github.com/facebookresearch/mvit/blob/19786631e330df9f3622e5402b4a419a263a2c80/mvit/models/attention.py # noqa B950
+ Args:
+ attn (Tensor): attention map.
+ q (Tensor): query q in the attention layer with shape (B, q_h * q_w, C).
+ rel_pos_h (Tensor): relative position embeddings (Lh, C) for height axis.
+ rel_pos_w (Tensor): relative position embeddings (Lw, C) for width axis.
+ q_size (Tuple): spatial sequence size of query q with (q_h, q_w).
+ k_size (Tuple): spatial sequence size of key k with (k_h, k_w).
+
+ Returns:
+ attn (Tensor): attention map with added relative positional embeddings.
+ """
+ q_h, q_w = q_size
+ k_h, k_w = k_size
+ Rh = get_rel_pos(q_h, k_h, rel_pos_h)
+ Rw = get_rel_pos(q_w, k_w, rel_pos_w)
+
+ B, _, dim = q.shape
+ r_q = q.reshape([B, q_h, q_w, dim])
+ rel_h = paddle.einsum("bhwc,hkc->bhwk", r_q, Rh)
+ rel_w = paddle.einsum("bhwc,wkc->bhwk", r_q, Rw)
+
+ attn = (attn.reshape([B, q_h, q_w, k_h, k_w]) + rel_h[:, :, :, :, None] +
+ rel_w[:, :, :, None, :]).reshape([B, q_h * q_w, k_h * k_w])
+
+ return attn
+
+
+class PatchEmbed(nn.Layer):
+ """
+ Image to Patch Embedding.
+ """
+
+ def __init__(
+ self,
+ kernel_size: Tuple[int, int]=(16, 16),
+ stride: Tuple[int, int]=(16, 16),
+ padding: Tuple[int, int]=(0, 0),
+ in_chans: int=3,
+ embed_dim: int=768, ) -> None:
+ """
+ Args:
+ kernel_size (Tuple): kernel size of the projection layer.
+ stride (Tuple): stride of the projection layer.
+ padding (Tuple): padding size of the projection layer.
+ in_chans (int): Number of input image channels.
+ embed_dim (int): embed_dim (int): Patch embedding dimension.
+ """
+ super().__init__()
+
+ self.proj = nn.Conv2D(
+ in_chans,
+ embed_dim,
+ kernel_size=kernel_size,
+ stride=stride,
+ padding=padding)
+
+ def forward(self, x: paddle.Tensor) -> paddle.Tensor:
+ x = self.proj(x)
+ # B C H W -> B H W C
+ x = paddle.transpose(x, [0, 2, 3, 1])
+ return x
+
+
+if __name__ == '__main__':
+ import numpy as np
+ import paddle
+ from padiff import auto_diff
+ import torch
+ from segment_anything.modeling import ImageEncoderViT as ImageEncoderViT_torch
+
+ # Set random seed
+ # np.random.seed(42)
+ paddle.set_device('gpu')
+ image_encoder_t = ImageEncoderViT_torch(
+ depth=12,
+ embed_dim=768,
+ img_size=1024,
+ mlp_ratio=4,
+ norm_layer=partial(
+ torch.nn.LayerNorm, eps=1e-6),
+ num_heads=12,
+ patch_size=16,
+ qkv_bias=True,
+ use_rel_pos=True,
+ global_attn_indexes=[2, 5, 8, 11],
+ window_size=14,
+ out_chans=256, )
+ # image_encoder_t = image_encoder_t.to('cuda:1')
+
+ image_encoder = ImageEncoderViT(
+ depth=12,
+ embed_dim=768,
+ img_size=1024,
+ mlp_ratio=4,
+ norm_layer=partial(
+ paddle.nn.LayerNorm, epsilon=1e-6),
+ num_heads=12,
+ patch_size=16,
+ qkv_bias=True,
+ use_rel_pos=True,
+ global_attn_indexes=[2, 5, 8, 11],
+ window_size=14,
+ out_chans=256, )
+
+ # Generate random numbers of shape (4, 3, 128, 128)
+ random_numbers = np.random.rand(1, 3, 1024, 1024).astype("float32")
+ inp = ({
+ 'x': paddle.to_tensor(random_numbers)
+ }, {
+ 'x': torch.as_tensor(random_numbers)
+ })
+
+ auto_diff(
+ image_encoder,
+ image_encoder_t,
+ inp,
+ auto_weights=True,
+ options={
+ 'atol': 0.001,
+ 'rtol': 0,
+ 'compare_mode': 'mean',
+ 'single_step': False
+ })
diff --git a/contrib/SegmentAnything/segment_anything/modeling/mask_decoder.py b/contrib/SegmentAnything/segment_anything/modeling/mask_decoder.py
new file mode 100644
index 0000000000..5766b6a191
--- /dev/null
+++ b/contrib/SegmentAnything/segment_anything/modeling/mask_decoder.py
@@ -0,0 +1,176 @@
+# Copyright (c) 2023 PaddlePaddle Authors. All Rights Reserved.
+#
+# Licensed under the Apache License, Version 2.0 (the "License");
+# you may not use this file except in compliance with the License.
+# You may obtain a copy of the License at
+#
+# http://www.apache.org/licenses/LICENSE-2.0
+#
+# Unless required by applicable law or agreed to in writing, software
+# distributed under the License is distributed on an "AS IS" BASIS,
+# WITHOUT WARRANTIES OR CONDITIONS OF ANY KIND, either express or implied.
+# See the License for the specific language governing permissions and
+# limitations under the License.
+
+# This implementation refers to: https://github.com/facebookresearch/segment-anything
+
+import paddle
+from typing import List, Tuple, Type
+from common import LayerNorm2d
+
+
+class MaskDecoder(paddle.nn.Layer):
+ def __init__(self,
+ *,
+ transformer_dim: int,
+ transformer: paddle.nn.Layer,
+ num_multimask_outputs: int=3,
+ activation: Type[paddle.nn.Layer]=paddle.nn.GELU,
+ iou_head_depth: int=3,
+ iou_head_hidden_dim: int=256) -> None:
+ """
+ Predicts masks given an image and prompt embeddings, using a
+ tranformer architecture.
+
+ Arguments:
+ transformer_dim (int): the channel dimension of the transformer
+ transformer (nn.Module): the transformer used to predict masks
+ num_multimask_outputs (int): the number of masks to predict
+ when disambiguating masks
+ activation (nn.Module): the type of activation to use when
+ upscaling masks
+ iou_head_depth (int): the depth of the MLP used to predict
+ mask quality
+ iou_head_hidden_dim (int): the hidden dimension of the MLP
+ used to predict mask quality
+ """
+ super().__init__()
+ self.transformer_dim = transformer_dim
+ self.transformer = transformer
+ self.num_multimask_outputs = num_multimask_outputs
+ self.iou_token = paddle.nn.Embedding(1, transformer_dim)
+ self.num_mask_tokens = num_multimask_outputs + 1
+ self.mask_tokens = paddle.nn.Embedding(self.num_mask_tokens,
+ transformer_dim)
+ self.output_upscaling = paddle.nn.Sequential(
+ paddle.nn.Conv2DTranspose(
+ in_channels=transformer_dim,
+ out_channels=transformer_dim // 4,
+ kernel_size=2,
+ stride=2),
+ LayerNorm2d(transformer_dim // 4),
+ activation(),
+ paddle.nn.Conv2DTranspose(
+ in_channels=transformer_dim // 4,
+ out_channels=transformer_dim // 8,
+ kernel_size=2,
+ stride=2),
+ activation())
+ self.output_hypernetworks_mlps = paddle.nn.LayerList(sublayers=[
+ MLP(transformer_dim, transformer_dim, transformer_dim // 8, 3)
+ for i in range(self.num_mask_tokens)
+ ])
+ self.iou_prediction_head = MLP(transformer_dim, iou_head_hidden_dim,
+ self.num_mask_tokens, iou_head_depth)
+
+ def forward(self,
+ image_embeddings: paddle.Tensor,
+ image_pe: paddle.Tensor,
+ sparse_prompt_embeddings: paddle.Tensor,
+ dense_prompt_embeddings: paddle.Tensor,
+ multimask_output: bool) -> Tuple[paddle.Tensor, paddle.Tensor]:
+ """
+ Predict masks given image and prompt embeddings.
+
+ Arguments:
+ image_embeddings (torch.Tensor): the embeddings from the image encoder
+ image_pe (torch.Tensor): positional encoding with the shape of image_embeddings
+ sparse_prompt_embeddings (torch.Tensor): the embeddings of the points and boxes
+ dense_prompt_embeddings (torch.Tensor): the embeddings of the mask inputs
+ multimask_output (bool): Whether to return multiple masks or a single
+ mask.
+
+ Returns:
+ torch.Tensor: batched predicted masks
+ torch.Tensor: batched predictions of mask quality
+ """
+ masks, iou_pred = self.predict_masks(
+ image_embeddings=image_embeddings,
+ image_pe=image_pe,
+ sparse_prompt_embeddings=sparse_prompt_embeddings,
+ dense_prompt_embeddings=dense_prompt_embeddings)
+
+ if multimask_output:
+ mask_slice = slice(1, None)
+ else:
+ mask_slice = slice(0, 1)
+ masks = masks[:, mask_slice, :, :]
+ iou_pred = iou_pred[:, mask_slice]
+
+ return masks, iou_pred
+
+ def predict_masks(self,
+ image_embeddings: paddle.Tensor,
+ image_pe: paddle.Tensor,
+ sparse_prompt_embeddings: paddle.Tensor,
+ dense_prompt_embeddings: paddle.Tensor) -> Tuple[
+ paddle.Tensor, paddle.Tensor]:
+ """Predicts masks. See 'forward' for more details."""
+ output_tokens = paddle.concat(
+ x=[self.iou_token.weight, self.mask_tokens.weight], axis=0)
+ output_tokens = output_tokens.unsqueeze(axis=0).expand(
+ shape=[sparse_prompt_embeddings.shape[0], -1, -1])
+ tokens = paddle.concat(
+ x=(output_tokens, sparse_prompt_embeddings), axis=1)
+ src = paddle.repeat_interleave(
+ image_embeddings, tokens.shape[0], axis=0)
+ src = src + dense_prompt_embeddings
+ pos_src = paddle.repeat_interleave(image_pe, tokens.shape[0], axis=0)
+ b, c, h, w = src.shape
+ hs, src = self.transformer(src, pos_src, tokens)
+
+ iou_token_out = hs[:, (0), :]
+ mask_tokens_out = hs[:, 1:1 + self.num_mask_tokens, :]
+ x = src
+ perm_0 = list(range(x.ndim))
+ perm_0[1] = 2
+ perm_0[2] = 1
+ """Class Method: *.view, not convert, please check whether it is torch.Tensor.*/Optimizer.*/nn.Module.*, and convert manually"""
+ src = x.transpose(perm=perm_0).reshape([b, c, h, w])
+ upscaled_embedding = self.output_upscaling(src)
+ hyper_in_list: List[paddle.Tensor] = []
+ for i in range(self.num_mask_tokens):
+ hyper_in_list.append(self.output_hypernetworks_mlps[i](
+ mask_tokens_out[:, (i), :]))
+ hyper_in = paddle.stack(x=hyper_in_list, axis=1)
+ b, c, h, w = upscaled_embedding.shape
+ """Class Method: *.view, not convert, please check whether it is torch.Tensor.*/Optimizer.*/nn.Module.*, and convert manually"""
+ """Class Method: *.view, not convert, please check whether it is torch.Tensor.*/Optimizer.*/nn.Module.*, and convert manually"""
+ masks = (hyper_in @upscaled_embedding.reshape([b, c, h * w])).reshape(
+ [b, -1, h, w])
+ iou_pred = self.iou_prediction_head(iou_token_out)
+ return masks, iou_pred
+
+
+class MLP(paddle.nn.Layer):
+ def __init__(self,
+ input_dim: int,
+ hidden_dim: int,
+ output_dim: int,
+ num_layers: int,
+ sigmoid_output: bool=False) -> None:
+ super().__init__()
+ self.num_layers = num_layers
+ h = [hidden_dim] * (num_layers - 1)
+ self.layers = paddle.nn.LayerList(sublayers=(paddle.nn.Linear(
+ in_features=n,
+ out_features=k) for n, k in zip([input_dim] + h, h + [output_dim])))
+ self.sigmoid_output = sigmoid_output
+
+ def forward(self, x):
+ for i, layer in enumerate(self.layers):
+ x = paddle.nn.functional.relu(
+ x=layer(x)) if i < self.num_layers - 1 else layer(x)
+ if self.sigmoid_output:
+ x = paddle.nn.functional.sigmoid(x=x)
+ return x
diff --git a/contrib/SegmentAnything/segment_anything/modeling/prompt_encoder.py b/contrib/SegmentAnything/segment_anything/modeling/prompt_encoder.py
new file mode 100644
index 0000000000..391052d7dc
--- /dev/null
+++ b/contrib/SegmentAnything/segment_anything/modeling/prompt_encoder.py
@@ -0,0 +1,238 @@
+# Copyright (c) 2023 PaddlePaddle Authors. All Rights Reserved.
+#
+# Licensed under the Apache License, Version 2.0 (the "License");
+# you may not use this file except in compliance with the License.
+# You may obtain a copy of the License at
+#
+# http://www.apache.org/licenses/LICENSE-2.0
+#
+# Unless required by applicable law or agreed to in writing, software
+# distributed under the License is distributed on an "AS IS" BASIS,
+# WITHOUT WARRANTIES OR CONDITIONS OF ANY KIND, either express or implied.
+# See the License for the specific language governing permissions and
+# limitations under the License.
+
+# This implementation refers to: https://github.com/facebookresearch/segment-anything
+
+import paddle
+import numpy as np
+from typing import Any, Optional, Tuple, Type
+from common import LayerNorm2d
+
+
+class PromptEncoder(paddle.nn.Layer):
+ def __init__(self,
+ embed_dim: int,
+ image_embedding_size: Tuple[int, int],
+ input_image_size: Tuple[int, int],
+ mask_in_chans: int,
+ activation: Type[paddle.nn.Layer]=paddle.nn.GELU) -> None:
+ """
+ Encodes prompts for input to SAM's mask decoder.
+
+ Arguments:
+ embed_dim (int): The prompts' embedding dimension
+ image_embedding_size (tuple(int, int)): The spatial size of the
+ image embedding, as (H, W).
+ input_image_size (int): The padded size of the image as input
+ to the image encoder, as (H, W).
+ mask_in_chans (int): The number of hidden channels used for
+ encoding input masks.
+ activation (nn.Module): The activation to use when encoding
+ input masks.
+ """
+ super().__init__()
+ self.embed_dim = embed_dim
+ self.input_image_size = input_image_size
+ self.image_embedding_size = image_embedding_size
+ self.pe_layer = PositionEmbeddingRandom(embed_dim // 2)
+ self.num_point_embeddings: int = 4
+ point_embeddings = [
+ paddle.nn.Embedding(1, embed_dim)
+ for i in range(self.num_point_embeddings)
+ ]
+ self.point_embeddings = paddle.nn.LayerList(sublayers=point_embeddings)
+ self.not_a_point_embed = paddle.nn.Embedding(1, embed_dim)
+ self.mask_input_size = 4 * image_embedding_size[
+ 0], 4 * image_embedding_size[1]
+ self.mask_downscaling = paddle.nn.Sequential(
+ paddle.nn.Conv2D(
+ in_channels=1,
+ out_channels=mask_in_chans // 4,
+ kernel_size=2,
+ stride=2),
+ LayerNorm2d(mask_in_chans // 4),
+ activation(),
+ paddle.nn.Conv2D(
+ in_channels=mask_in_chans // 4,
+ out_channels=mask_in_chans,
+ kernel_size=2,
+ stride=2),
+ LayerNorm2d(mask_in_chans),
+ activation(),
+ paddle.nn.Conv2D(
+ in_channels=mask_in_chans,
+ out_channels=embed_dim,
+ kernel_size=1))
+ self.no_mask_embed = paddle.nn.Embedding(1, embed_dim)
+
+ def get_dense_pe(self) -> paddle.Tensor:
+ """
+ Returns the positional encoding used to encode point prompts,
+ applied to a dense set of points the shape of the image encoding.
+
+ Returns:
+ torch.Tensor: Positional encoding with shape
+ 1x(embed_dim)x(embedding_h)x(embedding_w)
+ """
+ return self.pe_layer(self.image_embedding_size).unsqueeze(axis=0)
+
+ def _embed_points(self,
+ points: paddle.Tensor,
+ labels: paddle.Tensor,
+ pad: bool) -> paddle.Tensor:
+ """Embeds point prompts."""
+ points = points + 0.5
+ points = points.cast('float32')
+ if pad:
+ padding_point = paddle.zeros(shape=(points.shape[0], 1, 2))
+ padding_label = -paddle.ones(shape=(labels.shape[0], 1))
+ points = paddle.concat(x=[points, padding_point], axis=1)
+ labels = paddle.concat(
+ x=[labels.astype("float32"), padding_label], axis=1)
+ point_embedding = self.pe_layer.forward_with_coords(
+ points, self.input_image_size)
+
+ point_embedding[labels == -1] = 0.0
+ if point_embedding[labels == -1].shape[0] != 0:
+ point_embedding[labels == -1] += self.not_a_point_embed.weight
+ if point_embedding[labels == 0].shape[0] != 0:
+ point_embedding[labels == 0] += self.point_embeddings[0].weight
+ if point_embedding[labels == 1].shape[0] != 0:
+ point_embedding[labels == 1] += self.point_embeddings[1].weight
+ return point_embedding
+
+ def _embed_boxes(self, boxes: paddle.Tensor) -> paddle.Tensor:
+ """Embeds box prompts."""
+ boxes = boxes + 0.5
+ """Class Method: *.reshape, not convert, please check whether it is torch.Tensor.*/Optimizer.*/nn.Module.*, and convert manually"""
+ coords = boxes.reshape([-1, 2, 2])
+ corner_embedding = self.pe_layer.forward_with_coords(
+ coords, self.input_image_size)
+ corner_embedding[:, (0), :] += self.point_embeddings[2].weight
+ corner_embedding[:, (1), :] += self.point_embeddings[3].weight
+ return corner_embedding
+
+ def _embed_masks(self, masks: paddle.Tensor) -> paddle.Tensor:
+ """Embeds mask inputs."""
+ mask_embedding = self.mask_downscaling(masks)
+ return mask_embedding
+
+ def _get_batch_size(self,
+ points: Optional[Tuple[paddle.Tensor, paddle.Tensor]],
+ boxes: Optional[paddle.Tensor],
+ masks: Optional[paddle.Tensor]) -> int:
+ """
+ Gets the batch size of the output given the batch size of the input prompts.
+ """
+ if points is not None:
+ return points[0].shape[0]
+ elif boxes is not None:
+ return boxes.shape[0]
+ elif masks is not None:
+ return masks.shape[0]
+ else:
+ return 1
+
+ def _get_device(self):
+ return self.point_embeddings[0].weight.place
+
+ def forward(self,
+ points: Optional[Tuple[paddle.Tensor, paddle.Tensor]],
+ boxes: Optional[paddle.Tensor],
+ masks: Optional[paddle.Tensor]) -> Tuple[paddle.Tensor,
+ paddle.Tensor]:
+ """
+ Embeds different types of prompts, returning both sparse and dense
+ embeddings.
+
+ Arguments:
+ points (tuple(torch.Tensor, torch.Tensor) or none): point coordinates
+ and labels to embed.
+ boxes (torch.Tensor or none): boxes to embed
+ masks (torch.Tensor or none): masks to embed
+
+ Returns:
+ torch.Tensor: sparse embeddings for the points and boxes, with shape
+ BxNx(embed_dim), where N is determined by the number of input points
+ and boxes.
+ torch.Tensor: dense embeddings for the masks, in the shape
+ Bx(embed_dim)x(embed_H)x(embed_W)
+ """
+ bs = self._get_batch_size(points, boxes, masks)
+ sparse_embeddings = paddle.empty(shape=(bs, 0, self.embed_dim))
+ if points is not None:
+ coords, labels = points
+ point_embeddings = self._embed_points(
+ coords, labels, pad=boxes is None)
+ sparse_embeddings = paddle.concat(
+ x=[sparse_embeddings, point_embeddings], axis=1)
+ if boxes is not None:
+ box_embeddings = self._embed_boxes(boxes)
+ sparse_embeddings = paddle.concat(
+ x=[sparse_embeddings, box_embeddings], axis=1)
+ if masks is not None:
+ dense_embeddings = self._embed_masks(masks)
+ else:
+ """Class Method: *.reshape, not convert, please check whether it is torch.Tensor.*/Optimizer.*/nn.Module.*, and convert manually"""
+ dense_embeddings = self.no_mask_embed.weight.reshape(
+ [1, -1, 1, 1]).expand(shape=[
+ bs, -1, self.image_embedding_size[0],
+ self.image_embedding_size[1]
+ ])
+ return sparse_embeddings, dense_embeddings
+
+
+class PositionEmbeddingRandom(paddle.nn.Layer):
+ """
+ Positional encoding using random spatial frequencies.
+ """
+
+ def __init__(self, num_pos_feats: int=64,
+ scale: Optional[float]=None) -> None:
+ super().__init__()
+ if scale is None or scale <= 0.0:
+ scale = 1.0
+ self.register_buffer(
+ 'positional_encoding_gaussian_matrix',
+ scale * paddle.randn(shape=(2, num_pos_feats)))
+
+ def _pe_encoding(self, coords: paddle.Tensor) -> paddle.Tensor:
+ """Positionally encode points that are normalized to [0,1]."""
+ coords = 2 * coords - 1
+ coords = coords @self.positional_encoding_gaussian_matrix
+ coords = 2 * np.pi * coords
+ return paddle.concat(
+ x=[paddle.sin(x=coords), paddle.cos(x=coords)], axis=-1)
+
+ def forward(self, size: Tuple[int, int]) -> paddle.Tensor:
+ """Generate positional encoding for a grid of the specified size."""
+ h, w = size
+ device: Any = self.positional_encoding_gaussian_matrix.place
+ grid = paddle.ones(shape=(h, w), dtype='float32')
+ y_embed = grid.cumsum(axis=0) - 0.5
+ x_embed = grid.cumsum(axis=1) - 0.5
+ y_embed = y_embed / h
+ x_embed = x_embed / w
+ pe = self._pe_encoding(paddle.stack(x=[x_embed, y_embed], axis=-1))
+ return pe.transpose(perm=[2, 0, 1])
+
+ def forward_with_coords(self,
+ coords_input: paddle.Tensor,
+ image_size: Tuple[int, int]) -> paddle.Tensor:
+ """Positionally encode points that are not normalized to [0,1]."""
+ coords = coords_input.clone()
+ coords[:, :, (0)] = coords[:, :, (0)] / image_size[1]
+ coords[:, :, (1)] = coords[:, :, (1)] / image_size[0]
+ """Class Method: *.to, not convert, please check whether it is torch.Tensor.*/Optimizer.*/nn.Module.*, and convert manually"""
+ return self._pe_encoding(coords.astype('float32'))
diff --git a/contrib/SegmentAnything/segment_anything/modeling/sam.py b/contrib/SegmentAnything/segment_anything/modeling/sam.py
new file mode 100644
index 0000000000..ed4e6d8b60
--- /dev/null
+++ b/contrib/SegmentAnything/segment_anything/modeling/sam.py
@@ -0,0 +1,185 @@
+# Copyright (c) 2023 PaddlePaddle Authors. All Rights Reserved.
+#
+# Licensed under the Apache License, Version 2.0 (the "License");
+# you may not use this file except in compliance with the License.
+# You may obtain a copy of the License at
+#
+# http://www.apache.org/licenses/LICENSE-2.0
+#
+# Unless required by applicable law or agreed to in writing, software
+# distributed under the License is distributed on an "AS IS" BASIS,
+# WITHOUT WARRANTIES OR CONDITIONS OF ANY KIND, either express or implied.
+# See the License for the specific language governing permissions and
+# limitations under the License.
+
+# This implementation refers to: https://github.com/facebookresearch/segment-anything
+
+import paddle
+from paddle import nn
+from paddle.nn import functional as F
+
+from typing import Any, Dict, List, Tuple
+
+from .image_encoder import ImageEncoderViT
+from .mask_decoder import MaskDecoder
+from .prompt_encoder import PromptEncoder
+
+
+class Sam(nn.Layer):
+ mask_threshold: float = 0.0
+ image_format: str = "RGB"
+
+ def __init__(
+ self,
+ image_encoder: ImageEncoderViT,
+ prompt_encoder: PromptEncoder,
+ mask_decoder: MaskDecoder,
+ pixel_mean: List[float]=[123.675, 116.28, 103.53],
+ pixel_std: List[float]=[58.395, 57.12, 57.375], ) -> None:
+ """
+ SAM predicts object masks from an image and input prompts.
+
+ Arguments:
+ image_encoder (ImageEncoderViT): The backbone used to encode the
+ image into image embeddings that allow for efficient mask prediction.
+ prompt_encoder (PromptEncoder): Encodes various types of input prompts.
+ mask_decoder (MaskDecoder): Predicts masks from the image embeddings
+ and encoded prompts.
+ pixel_mean (list(float)): Mean values for normalizing pixels in the input image.
+ pixel_std (list(float)): Std values for normalizing pixels in the input image.
+ """
+ super().__init__()
+ self.image_encoder = image_encoder
+ self.prompt_encoder = prompt_encoder
+ self.mask_decoder = mask_decoder
+ self.register_buffer(
+ "pixel_mean",
+ paddle.to_tensor(pixel_mean).reshape([-1, 1, 1]),
+ persistable=False)
+ self.register_buffer(
+ "pixel_std",
+ paddle.to_tensor(pixel_std).reshape([-1, 1, 1]),
+ persistable=False)
+
+ @property
+ def device(self) -> Any:
+ return 'gpu'
+
+ @paddle.no_grad()
+ def forward(
+ self,
+ batched_input: List[Dict[str, Any]],
+ multimask_output: bool, ) -> List[Dict[str, paddle.Tensor]]:
+ """
+ Predicts masks end-to-end from provided images and prompts.
+ If prompts are not known in advance, using SamPredictor is
+ recommended over calling the model directly.
+
+ Arguments:
+ batched_input (list(dict)): A list over input images, each a
+ dictionary with the following keys. A prompt key can be
+ excluded if it is not present.
+ 'image': The image as a paddle tensor in 3xHxW format,
+ already transformed for input to the model.
+ 'original_size': (tuple(int, int)) The original size of
+ the image before transformation, as (H, W).
+ 'point_coords': (paddle.Tensor) Batched point prompts for
+ this image, with shape BxNx2. Already transformed to the
+ input frame of the model.
+ 'point_labels': (paddle.Tensor) Batched labels for point prompts,
+ with shape BxN.
+ 'boxes': (paddle.Tensor) Batched box inputs, with shape Bx4.
+ Already transformed to the input frame of the model.
+ 'mask_inputs': (paddle.Tensor) Batched mask inputs to the model,
+ in the form Bx1xHxW.
+ multimask_output (bool): Whether the model should predict multiple
+ disambiguating masks, or return a single mask.
+
+ Returns:
+ (list(dict)): A list over input images, where each element is
+ as dictionary with the following keys.
+ 'masks': (paddle.Tensor) Batched binary mask predictions,
+ with shape BxCxHxW, where B is the number of input promts,
+ C is determiend by multimask_output, and (H, W) is the
+ original size of the image.
+ 'iou_predictions': (paddle.Tensor) The model's predictions
+ of mask quality, in shape BxC.
+ 'low_res_logits': (paddle.Tensor) Low resolution logits with
+ shape BxCxHxW, where H=W=256. Can be passed as mask input
+ to subsequent iterations of prediction.
+ """
+ input_images = paddle.stack(
+ [self.preprocess(x["image"]) for x in batched_input], axis=0)
+ image_embeddings = self.image_encoder(input_images)
+
+ outputs = []
+ for image_record, curr_embedding in zip(batched_input,
+ image_embeddings):
+ if "point_coords" in image_record:
+ points = (image_record["point_coords"],
+ image_record["point_labels"])
+ else:
+ points = None
+ sparse_embeddings, dense_embeddings = self.prompt_encoder(
+ points=points,
+ boxes=image_record.get("boxes", None),
+ masks=image_record.get("mask_inputs", None), )
+ low_res_masks, iou_predictions = self.mask_decoder(
+ image_embeddings=curr_embedding.unsqueeze(0),
+ image_pe=self.prompt_encoder.get_dense_pe(),
+ sparse_prompt_embeddings=sparse_embeddings,
+ dense_prompt_embeddings=dense_embeddings,
+ multimask_output=multimask_output, )
+ masks = self.postprocess_masks(
+ low_res_masks,
+ input_size=image_record["image"].shape[-2:],
+ original_size=image_record["original_size"], )
+ masks = masks > self.mask_threshold
+ outputs.append({
+ "masks": masks,
+ "iou_predictions": iou_predictions,
+ "low_res_logits": low_res_masks,
+ })
+ return outputs
+
+ def postprocess_masks(
+ self,
+ masks: paddle.Tensor,
+ input_size: Tuple[int, ...],
+ original_size: Tuple[int, ...], ) -> paddle.Tensor:
+ """
+ Remove padding and upscale masks to the original image size.
+
+ Arguments:
+ masks (paddle.Tensor): Batched masks from the mask_decoder,
+ in BxCxHxW format.
+ input_size (tuple(int, int)): The size of the image input to the
+ model, in (H, W) format. Used to remove padding.
+ original_size (tuple(int, int)): The original size of the image
+ before resizing for input to the model, in (H, W) format.
+
+ Returns:
+ (paddle.Tensor): Batched masks in BxCxHxW format, where (H, W)
+ is given by original_size.
+ """
+ masks = F.interpolate(
+ masks,
+ (self.image_encoder.img_size, self.image_encoder.img_size),
+ mode="bilinear",
+ align_corners=False, )
+ masks = masks[..., :input_size[0], :input_size[1]]
+ masks = F.interpolate(
+ masks, original_size, mode="bilinear", align_corners=False)
+ return masks
+
+ def preprocess(self, x: paddle.Tensor) -> paddle.Tensor:
+ """Normalize pixel values and pad to a square input."""
+ # Normalize colors
+ x = (x - self.pixel_mean) / self.pixel_std
+
+ # Pad
+ h, w = x.shape[-2:]
+ padh = self.image_encoder.img_size - h
+ padw = self.image_encoder.img_size - w
+ x = F.pad(x, (0, padw, 0, padh))
+ return x
diff --git a/contrib/SegmentAnything/segment_anything/modeling/transformer.py b/contrib/SegmentAnything/segment_anything/modeling/transformer.py
new file mode 100644
index 0000000000..0ea1f43b55
--- /dev/null
+++ b/contrib/SegmentAnything/segment_anything/modeling/transformer.py
@@ -0,0 +1,200 @@
+# Copyright (c) 2023 PaddlePaddle Authors. All Rights Reserved.
+#
+# Licensed under the Apache License, Version 2.0 (the "License");
+# you may not use this file except in compliance with the License.
+# You may obtain a copy of the License at
+#
+# http://www.apache.org/licenses/LICENSE-2.0
+#
+# Unless required by applicable law or agreed to in writing, software
+# distributed under the License is distributed on an "AS IS" BASIS,
+# WITHOUT WARRANTIES OR CONDITIONS OF ANY KIND, either express or implied.
+# See the License for the specific language governing permissions and
+# limitations under the License.
+
+# This implementation refers to: https://github.com/facebookresearch/segment-anything
+
+import paddle
+from paddle import Tensor, nn
+import paddle.nn.functional as F
+import math
+from typing import Tuple, Type
+from common import MLPBlock
+
+
+class TwoWayTransformer(nn.Layer):
+ def __init__(self,
+ depth: int,
+ embedding_dim: int,
+ num_heads: int,
+ mlp_dim: int,
+ activation: Type[nn.Layer]=nn.ReLU,
+ attention_downsample_rate: int=2) -> None:
+ """
+ A transformer decoder that attends to an input image using
+ queries whose positional embedding is supplied.
+
+ Args:
+ depth (int): number of layers in the transformer
+ embedding_dim (int): the channel dimension for the input embeddings
+ num_heads (int): the number of heads for multihead attention. Must
+ divide embedding_dim
+ mlp_dim (int): the channel dimension internal to the MLP block
+ activation (nn.Layer): the activation to use in the MLP block
+ """
+ super().__init__()
+ self.depth = depth
+ self.embedding_dim = embedding_dim
+ self.num_heads = num_heads
+ self.mlp_dim = mlp_dim
+ self.layers = nn.LayerList()
+ for i in range(depth):
+ self.layers.append(
+ TwoWayAttentionBlock(
+ embedding_dim=embedding_dim,
+ num_heads=num_heads,
+ mlp_dim=mlp_dim,
+ activation=activation,
+ attention_downsample_rate=attention_downsample_rate,
+ skip_first_layer_pe=i == 0))
+ self.final_attn_token_to_image = Attention(
+ embedding_dim, num_heads, downsample_rate=attention_downsample_rate)
+ self.norm_final_attn = nn.LayerNorm(embedding_dim)
+
+ def forward(self, image_embedding, image_pe,
+ point_embedding) -> Tuple[Tensor, Tensor]:
+ """
+ Args:
+ image_embedding (paddle.Tensor): image to attend to. Should be shape
+ B x embedding_dim x h x w for any h and w.
+ image_pe (paddle.Tensor): the positional encoding to add to the image. Must
+ have the same shape as image_embedding.
+ point_embedding (paddle.Tensor): the embedding to add to the query points.
+ Must have shape B x N_points x embedding_dim for any N_points.
+
+ Returns:
+ paddle.Tensor: the processed point_embedding
+ paddle.Tensor: the processed image_embedding
+ """
+ bs, c, h, w = image_embedding.shape
+ image_embedding = image_embedding.flatten(2).transpose([0, 2, 1])
+ image_pe = image_pe.flatten(2).transpose([0, 2, 1])
+ queries = point_embedding
+ keys = image_embedding
+ for layer in self.layers:
+ queries, keys = layer(
+ queries=queries,
+ keys=keys,
+ query_pe=point_embedding,
+ key_pe=image_pe)
+ q = queries + point_embedding
+ k = keys + image_pe
+ attn_out = self.final_attn_token_to_image(q=q, k=k, v=keys)
+ queries = queries + attn_out
+ queries = self.norm_final_attn(queries)
+ return queries, keys
+
+
+class TwoWayAttentionBlock(nn.Layer):
+ def __init__(self,
+ embedding_dim: int,
+ num_heads: int,
+ mlp_dim: int=2048,
+ activation: Type[nn.Layer]=nn.ReLU,
+ attention_downsample_rate: int=2,
+ skip_first_layer_pe: bool=False) -> None:
+ """
+ A transformer block with four layers: (1) self-attention of sparse
+ inputs, (2) cross attention of sparse inputs to dense inputs, (3) mlp
+ block on sparse inputs, and (4) cross attention of dense inputs to sparse
+ inputs.
+
+ Arguments:
+ embedding_dim (int): the channel dimension of the embeddings
+ num_heads (int): the number of heads in the attention layers
+ mlp_dim (int): the hidden dimension of the mlp block
+ activation (nn.Layer): the activation of the mlp block
+ skip_first_layer_pe (bool): skip the PE on the first layer
+ """
+ super().__init__()
+ self.self_attn = Attention(embedding_dim, num_heads)
+ self.norm1 = nn.LayerNorm(embedding_dim)
+ self.cross_attn_token_to_image = Attention(
+ embedding_dim, num_heads, downsample_rate=attention_downsample_rate)
+ self.norm2 = nn.LayerNorm(embedding_dim)
+ self.mlp = MLPBlock(embedding_dim, mlp_dim, activation)
+ self.norm3 = nn.LayerNorm(embedding_dim)
+ self.norm4 = nn.LayerNorm(embedding_dim)
+ self.cross_attn_image_to_token = Attention(
+ embedding_dim, num_heads, downsample_rate=attention_downsample_rate)
+ self.skip_first_layer_pe = skip_first_layer_pe
+
+ def forward(self, queries, keys, query_pe, key_pe) -> Tuple[Tensor, Tensor]:
+ if self.skip_first_layer_pe:
+ queries = self.self_attn(q=queries, k=queries, v=queries)
+ else:
+ q = queries + query_pe
+ attn_out = self.self_attn(q=q, k=q, v=queries)
+ queries = queries + attn_out
+ queries = self.norm1(queries)
+ q = queries + query_pe
+ k = keys + key_pe
+ attn_out = self.cross_attn_token_to_image(q=q, k=k, v=keys)
+ queries = queries + attn_out
+ queries = self.norm2(queries)
+ mlp_out = self.mlp(queries)
+ queries = queries + mlp_out
+ queries = self.norm3(queries)
+ q = queries + query_pe
+ k = keys + key_pe
+ attn_out = self.cross_attn_image_to_token(q=k, k=q, v=queries)
+ keys = keys + attn_out
+ keys = self.norm4(keys)
+ return queries, keys
+
+
+class Attention(nn.Layer):
+ """
+ An attention layer that allows for downscaling the size of the embedding
+ after projection to queries, keys, and values.
+ """
+
+ def __init__(self,
+ embedding_dim: int,
+ num_heads: int,
+ downsample_rate: int=1) -> None:
+ super().__init__()
+ self.embedding_dim = embedding_dim
+ self.internal_dim = embedding_dim // downsample_rate
+ self.num_heads = num_heads
+ assert self.internal_dim % num_heads == 0, 'num_heads must divide embedding_dim.'
+ self.q_proj = nn.Linear(embedding_dim, self.internal_dim)
+ self.k_proj = nn.Linear(embedding_dim, self.internal_dim)
+ self.v_proj = nn.Linear(embedding_dim, self.internal_dim)
+ self.out_proj = nn.Linear(self.internal_dim, embedding_dim)
+
+ def _separate_heads(self, x, num_heads: int):
+ b, n, c = x.shape
+ x = x.reshape([b, n, num_heads, c // num_heads])
+ return x.transpose([0, 2, 1, 3])
+
+ def _recombine_heads(self, x):
+ b, n_heads, n_tokens, c_per_head = x.shape
+ x = x.transpose([0, 2, 1, 3])
+ return x.reshape([b, n_tokens, n_heads * c_per_head])
+
+ def forward(self, q, k, v):
+ q = self.q_proj(q)
+ k = self.k_proj(k)
+ v = self.v_proj(v)
+ q = self._separate_heads(q, self.num_heads)
+ k = self._separate_heads(k, self.num_heads)
+ v = self._separate_heads(v, self.num_heads)
+ _, _, _, c_per_head = q.shape
+ attn = q @k.transpose([0, 1, 3, 2])
+ attn = attn / math.sqrt(c_per_head)
+ attn = F.softmax(attn, axis=-1)
+ out = attn @v
+ out = self._recombine_heads(out)
+ out = self.out_proj(out)
+ return out
diff --git a/contrib/SegmentAnything/segment_anything/predictor.py b/contrib/SegmentAnything/segment_anything/predictor.py
new file mode 100644
index 0000000000..641dfec16f
--- /dev/null
+++ b/contrib/SegmentAnything/segment_anything/predictor.py
@@ -0,0 +1,282 @@
+# Copyright (c) 2023 PaddlePaddle Authors. All Rights Reserved.
+#
+# Licensed under the Apache License, Version 2.0 (the "License");
+# you may not use this file except in compliance with the License.
+# You may obtain a copy of the License at
+#
+# http://www.apache.org/licenses/LICENSE-2.0
+#
+# Unless required by applicable law or agreed to in writing, software
+# distributed under the License is distributed on an "AS IS" BASIS,
+# WITHOUT WARRANTIES OR CONDITIONS OF ANY KIND, either express or implied.
+# See the License for the specific language governing permissions and
+# limitations under the License.
+
+# This implementation refers to: https://github.com/facebookresearch/segment-anything
+
+import numpy as np
+from typing import Optional, Tuple
+
+import paddle
+
+from .modeling import Sam
+from .utils.transforms import ResizeLongestSide
+
+
+class SamPredictor:
+ def __init__(
+ self,
+ sam_model: Sam, ) -> None:
+ """
+ Uses SAM to calculate the image embedding for an image, and then
+ allow repeated, efficient mask prediction given prompts.
+
+ Arguments:
+ sam_model (Sam): The model to use for mask prediction.
+ """
+ super().__init__()
+ self.model = sam_model
+ self.transform = ResizeLongestSide(sam_model.image_encoder.img_size)
+ self.reset_image()
+
+ def set_image(
+ self,
+ image: np.ndarray,
+ image_format: str="RGB", ) -> None:
+ """
+ Calculates the image embeddings for the provided image, allowing
+ masks to be predicted with the 'predict' method.
+
+ Arguments:
+ image (np.ndarray): The image for calculating masks. Expects an
+ image in HWC uint8 format, with pixel values in [0, 255].
+ image_format (str): The color format of the image, in ['RGB', 'BGR'].
+ """
+ assert image_format in [
+ "RGB",
+ "BGR",
+ ], f"image_format must be in ['RGB', 'BGR'], is {image_format}."
+ if image_format != self.model.image_format:
+ image = image[..., ::-1]
+
+ # Transform the image to the form expected by the model
+ input_image = self.transform.apply_image(image) # numpy array
+ input_image_paddle = paddle.to_tensor(input_image).cast('int32')
+
+ input_image_paddle = input_image_paddle.transpose(
+ [2, 0, 1])[None, :, :, :]
+
+ self.set_paddle_image(input_image_paddle, image.shape[:2])
+
+ @paddle.no_grad()
+ def set_paddle_image(
+ self,
+ transformed_image: paddle.Tensor,
+ original_image_size: Tuple[int, ...], ) -> None:
+ """
+ Calculates the image embeddings for the provided image, allowing
+ masks to be predicted with the 'predict' method. Expects the input
+ image to be already transformed to the format expected by the model.
+
+ Arguments:
+ transformed_image (paddle.Tensor): The input image, with shape
+ 1x3xHxW, which has been transformed with ResizeLongestSide.
+ original_image_size (tuple(int, int)): The size of the image
+ before transformation, in (H, W) format.
+ """
+ assert (
+ len(transformed_image.shape) == 4 and
+ transformed_image.shape[1] == 3 and
+ max(*transformed_image.shape[2:]) ==
+ self.model.image_encoder.img_size
+ ), f"set_paddle_image input must be BCHW with long side {self.model.image_encoder.img_size}."
+ self.reset_image()
+
+ self.original_size = original_image_size
+ self.input_size = tuple(transformed_image.shape[-2:])
+ input_image = self.model.preprocess(transformed_image)
+ self.features = self.model.image_encoder(input_image)
+ self.is_image_set = True
+
+ def predict(
+ self,
+ point_coords: Optional[np.ndarray]=None,
+ point_labels: Optional[np.ndarray]=None,
+ box: Optional[np.ndarray]=None,
+ mask_input: Optional[np.ndarray]=None,
+ multimask_output: bool=True,
+ return_logits: bool=False, ) -> Tuple[paddle.Tensor, paddle.Tensor,
+ paddle.Tensor]:
+ """
+ Predict masks for the given input prompts, using the currently set image.
+
+ Arguments:
+ point_coords (np.ndarray or None): A Nx2 array of point prompts to the
+ model. Each point is in (X,Y) in pixels.
+ point_labels (np.ndarray or None): A length N array of labels for the
+ point prompts. 1 indicates a foreground point and 0 indicates a
+ background point.
+ box (np.ndarray or None): A length 4 array given a box prompt to the
+ model, in XYXY format.
+ mask_input (np.ndarray): A low resolution mask input to the model, typically
+ coming from a previous prediction iteration. Has form 1xHxW, where
+ for SAM, H=W=256.
+ multimask_output (bool): If true, the model will return three masks.
+ For ambiguous input prompts (such as a single click), this will often
+ produce better masks than a single prediction. If only a single
+ mask is needed, the model's predicted quality score can be used
+ to select the best mask. For non-ambiguous prompts, such as multiple
+ input prompts, multimask_output=False can give better results.
+ return_logits (bool): If true, returns un-thresholded masks logits
+ instead of a binary mask.
+
+ Returns:
+ (np.ndarray): The output masks in CxHxW format, where C is the
+ number of masks, and (H, W) is the original image size.
+ (np.ndarray): An array of length C containing the model's
+ predictions for the quality of each mask.
+ (np.ndarray): An array of shape CxHxW, where C is the number
+ of masks and H=W=256. These low resolution logits can be passed to
+ a subsequent iteration as mask input.
+ """
+ if not self.is_image_set:
+ raise RuntimeError(
+ "An image must be set with .set_image(...) before mask prediction."
+ )
+
+ # Transform input prompts
+ coords_paddle, labels_paddle, box_paddle, mask_input_paddle = None, None, None, None
+ if point_coords is not None:
+ assert (
+ point_labels is not None
+ ), "point_labels must be supplied if point_coords is supplied."
+ point_coords = self.transform.apply_coords(point_coords,
+ self.original_size)
+ coords_paddle = paddle.to_tensor(point_coords).cast('float32')
+ labels_paddle = paddle.to_tensor(point_labels).cast('int32')
+ coords_paddle, labels_paddle = coords_paddle[
+ None, :, :], labels_paddle[None, :]
+ if box is not None:
+ box = self.transform.apply_boxes(box, self.original_size)
+ box_paddle = paddle.to_tensor(box).cast('float32')
+ box_paddle = box_paddle[None, :]
+ if mask_input is not None:
+ mask_input_paddle = paddle.to_tensor(mask_input).cast('float32')
+ mask_input_paddle = mask_input_paddle[None, :, :, :]
+
+ masks, iou_predictions, low_res_masks = self.predict_paddle(
+ coords_paddle,
+ labels_paddle,
+ box_paddle,
+ mask_input_paddle,
+ multimask_output,
+ return_logits=return_logits, )
+
+ masks = masks[0].detach().cpu().numpy()
+ iou_predictions = iou_predictions[0].detach().cpu().numpy()
+ low_res_masks = low_res_masks[0].detach().cpu().numpy()
+ return masks, iou_predictions, low_res_masks
+
+ @paddle.no_grad()
+ def predict_paddle(
+ self,
+ point_coords: Optional[paddle.Tensor],
+ point_labels: Optional[paddle.Tensor],
+ boxes: Optional[paddle.Tensor]=None,
+ mask_input: Optional[paddle.Tensor]=None,
+ multimask_output: bool=True,
+ return_logits: bool=False, ) -> Tuple[paddle.Tensor, paddle.Tensor,
+ paddle.Tensor]:
+ """
+ Predict masks for the given input prompts, using the currently set image.
+ Input prompts are batched paddle tensors and are expected to already be
+ transformed to the input frame using ResizeLongestSide.
+
+ Arguments:
+ point_coords (paddle.Tensor or None): A BxNx2 array of point prompts to the
+ model. Each point is in (X,Y) in pixels.
+ point_labels (paddle.Tensor or None): A BxN array of labels for the
+ point prompts. 1 indicates a foreground point and 0 indicates a
+ background point.
+ box (np.ndarray or None): A Bx4 array given a box prompt to the
+ model, in XYXY format.
+ mask_input (np.ndarray): A low resolution mask input to the model, typically
+ coming from a previous prediction iteration. Has form Bx1xHxW, where
+ for SAM, H=W=256. Masks returned by a previous iteration of the
+ predict method do not need further transformation.
+ multimask_output (bool): If true, the model will return three masks.
+ For ambiguous input prompts (such as a single click), this will often
+ produce better masks than a single prediction. If only a single
+ mask is needed, the model's predicted quality score can be used
+ to select the best mask. For non-ambiguous prompts, such as multiple
+ input prompts, multimask_output=False can give better results.
+ return_logits (bool): If true, returns un-thresholded masks logits
+ instead of a binary mask.
+
+ Returns:
+ (paddle.Tensor): The output masks in BxCxHxW format, where C is the
+ number of masks, and (H, W) is the original image size.
+ (paddle.Tensor): An array of shape BxC containing the model's
+ predictions for the quality of each mask.
+ (paddle.Tensor): An array of shape BxCxHxW, where C is the number
+ of masks and H=W=256. These low res logits can be passed to
+ a subsequent iteration as mask input.
+ """
+ if not self.is_image_set:
+ raise RuntimeError(
+ "An image must be set with .set_image(...) before mask prediction."
+ )
+
+ if point_coords is not None:
+ points = (point_coords, point_labels)
+ else:
+ points = None
+
+ # Embed prompts
+ sparse_embeddings, dense_embeddings = self.model.prompt_encoder(
+ points=points,
+ boxes=boxes,
+ masks=mask_input, )
+
+ # Predict masks
+ low_res_masks, iou_predictions = self.model.mask_decoder(
+ image_embeddings=self.features,
+ image_pe=self.model.prompt_encoder.get_dense_pe(),
+ sparse_prompt_embeddings=sparse_embeddings,
+ dense_prompt_embeddings=dense_embeddings,
+ multimask_output=multimask_output, )
+
+ # Upscale the masks to the original image resolution
+ masks = self.model.postprocess_masks(low_res_masks, self.input_size,
+ self.original_size)
+
+ if not return_logits:
+ masks = masks > self.model.mask_threshold
+
+ return masks, iou_predictions, low_res_masks
+
+ def get_image_embedding(self) -> paddle.Tensor:
+ """
+ Returns the image embeddings for the currently set image, with
+ shape 1xCxHxW, where C is the embedding dimension and (H,W) are
+ the embedding spatial dimension of SAM (typically C=256, H=W=64).
+ """
+ if not self.is_image_set:
+ raise RuntimeError(
+ "An image must be set with .set_image(...) to generate an embedding."
+ )
+ assert self.features is not None, "Features must exist if an image has been set."
+ return self.features
+
+ @property
+ def device(self) -> paddle.device:
+ return 'gpu'
+
+ def reset_image(self) -> None:
+ """Resets the currently set image."""
+ self.is_image_set = False
+ self.features = None
+ self.orig_h = None
+ self.orig_w = None
+ self.input_h = None
+ self.input_w = None
diff --git a/contrib/SegmentAnything/segment_anything/utils/__init__.py b/contrib/SegmentAnything/segment_anything/utils/__init__.py
new file mode 100644
index 0000000000..e69de29bb2
diff --git a/contrib/SegmentAnything/segment_anything/utils/transforms.py b/contrib/SegmentAnything/segment_anything/utils/transforms.py
new file mode 100644
index 0000000000..7308de0fb8
--- /dev/null
+++ b/contrib/SegmentAnything/segment_anything/utils/transforms.py
@@ -0,0 +1,229 @@
+# Copyright (c) 2023 PaddlePaddle Authors. All Rights Reserved.
+#
+# Licensed under the Apache License, Version 2.0 (the "License");
+# you may not use this file except in compliance with the License.
+# You may obtain a copy of the License at
+#
+# http://www.apache.org/licenses/LICENSE-2.0
+#
+# Unless required by applicable law or agreed to in writing, software
+# distributed under the License is distributed on an "AS IS" BASIS,
+# WITHOUT WARRANTIES OR CONDITIONS OF ANY KIND, either express or implied.
+# See the License for the specific language governing permissions and
+# limitations under the License.
+
+# This implementation refers to: https://github.com/facebookresearch/segment-anything
+
+import numpy as np
+import PIL
+from PIL import Image
+from copy import deepcopy
+from typing import Tuple
+
+import paddle
+from paddle.nn import functional as F
+from paddle.vision.transforms.functional import resize # type: ignore
+
+
+def to_pil_image(pic, mode=None):
+ """Convert a tensor or an ndarray to PIL Image.
+
+ Args:
+ pic (Tensor or numpy.ndarray): Image to be converted to PIL Image.
+ mode (`PIL.Image mode`_): color space and pixel depth of input data (optional).
+
+ .. _PIL.Image mode: https://pillow.readthedocs.io/en/latest/handbook/concepts.html#concept-modes
+
+ Returns:
+ PIL Image: Image converted to PIL Image.
+ """
+ if not (isinstance(pic, paddle.Tensor) or isinstance(pic, np.ndarray)):
+ raise TypeError(f"pic should be Tensor or ndarray. Got {type(pic)}.")
+
+ elif isinstance(pic, paddle.Tensor):
+ if pic.ndim not in {2, 3}:
+ raise ValueError(
+ f"pic should be 2/3 dimensional. Got {pic.ndim} dimensions.")
+
+ elif pic.ndim == 2:
+ # if 2D image, add channel dimension (CHW)
+ pic = pic.unsqueeze(0)
+
+ # check number of channels
+ if pic.shape[-3] > 4:
+ raise ValueError(
+ f"pic should not have > 4 channels. Got {pic.shape[-3]} channels."
+ )
+
+ elif isinstance(pic, np.ndarray):
+ if pic.ndim not in {2, 3}:
+ raise ValueError(
+ f"pic should be 2/3 dimensional. Got {pic.ndim} dimensions.")
+
+ elif pic.ndim == 2:
+ # if 2D image, add channel dimension (HWC)
+ pic = np.expand_dims(pic, 2)
+
+ # check number of channels
+ if pic.shape[-1] > 4:
+ raise ValueError(
+ f"pic should not have > 4 channels. Got {pic.shape[-1]} channels."
+ )
+
+ npimg = pic
+ if isinstance(pic, paddle.Tensor):
+ if pic.is_floating_point() and mode != "F":
+ pic = (pic * 255).astype("uint8")
+ npimg = np.transpose(pic.cpu().numpy(), (1, 2, 0))
+
+ if not isinstance(npimg, np.ndarray):
+ raise TypeError(
+ "Input pic must be a paddle.Tensor or NumPy ndarray, not {type(npimg)}"
+ )
+
+ if npimg.shape[2] == 1:
+ expected_mode = None
+ npimg = npimg[:, :, 0]
+ if npimg.dtype == np.uint8:
+ expected_mode = "L"
+ elif npimg.dtype == np.int16:
+ expected_mode = "I;16"
+ elif npimg.dtype == np.int32:
+ expected_mode = "I"
+ elif npimg.dtype == np.float32:
+ expected_mode = "F"
+ if mode is not None and mode != expected_mode:
+ raise ValueError(
+ f"Incorrect mode ({mode}) supplied "
+ f"for input type {np.dtype}. Should be {expected_mode}")
+ mode = expected_mode
+
+ elif npimg.shape[2] == 2:
+ permitted_2_channel_modes = ["LA"]
+ if mode is not None and mode not in permitted_2_channel_modes:
+ raise ValueError(
+ f"Only modes {permitted_2_channel_modes} are supported for 2D inputs"
+ )
+
+ if mode is None and npimg.dtype == np.uint8:
+ mode = "LA"
+
+ elif npimg.shape[2] == 4:
+ permitted_4_channel_modes = ["RGBA", "CMYK", "RGBX"]
+ if mode is not None and mode not in permitted_4_channel_modes:
+ raise ValueError(
+ f"Only modes {permitted_4_channel_modes} are supported for 4D inputs"
+ )
+
+ if mode is None and npimg.dtype == np.uint8:
+ mode = "RGBA"
+ else:
+ permitted_3_channel_modes = ["RGB", "YCbCr", "HSV"]
+ if mode is not None and mode not in permitted_3_channel_modes:
+ raise ValueError(
+ f"Only modes {permitted_3_channel_modes} are supported for 3D inputs"
+ )
+ if mode is None and npimg.dtype == np.uint8:
+ mode = "RGB"
+
+ if mode is None:
+ raise TypeError(f"Input type {npimg.dtype} is not supported")
+
+ return Image.fromarray(npimg, mode=mode)
+
+
+class ResizeLongestSide:
+ """
+ Resizes images to longest side 'target_length', as well as provides
+ methods for resizing coordinates and boxes. Provides methods for
+ transforming both numpy array and batched paddle tensors.
+ """
+
+ def __init__(self, target_length: int) -> None:
+ self.target_length = target_length
+
+ def apply_image(self, image: np.ndarray) -> np.ndarray:
+ """
+ Expects a numpy array with shape HxWxC in uint8 format.
+ """
+ target_size = self.get_preprocess_shape(image.shape[0], image.shape[1],
+ self.target_length)
+ return np.array(resize(to_pil_image(image), target_size))
+
+ def apply_coords(self, coords: np.ndarray,
+ original_size: Tuple[int, ...]) -> np.ndarray:
+ """
+ Expects a numpy array of length 2 in the final dimension. Requires the
+ original image size in (H, W) format.
+ """
+ old_h, old_w = original_size
+ new_h, new_w = self.get_preprocess_shape(
+ original_size[0], original_size[1], self.target_length)
+ coords = deepcopy(coords).astype(float)
+ coords[..., 0] = coords[..., 0] * (new_w / old_w)
+ coords[..., 1] = coords[..., 1] * (new_h / old_h)
+ return coords
+
+ def apply_boxes(self, boxes: np.ndarray,
+ original_size: Tuple[int, ...]) -> np.ndarray:
+ """
+ Expects a numpy array shape Bx4. Requires the original image size
+ in (H, W) format.
+ """
+ boxes = self.apply_coords(boxes.reshape([-1, 2, 2]), original_size)
+ return boxes.reshape([-1, 4])
+
+ def apply_image_paddle(self,
+ image: paddle.Tensor) -> paddle.Tensor: # not used
+ """
+ Expects batched images with shape BxCxHxW and float format. This
+ transformation may not exactly match apply_image. apply_image is
+ the transformation expected by the model.
+ """
+ # Expects an image in BCHW format. May not exactly match apply_image.
+ target_size = self.get_preprocess_shape(image.shape[0], image.shape[1],
+ self.target_length)
+ return F.interpolate(
+ image,
+ target_size,
+ mode="bilinear",
+ align_corners=False, # todo: missing this flag antialias=True
+ )
+
+ def apply_coords_paddle(self,
+ coords: paddle.Tensor,
+ original_size: Tuple[int, ...]) -> paddle.Tensor:
+ """
+ Expects a paddle tensor with length 2 in the last dimension. Requires the
+ original image size in (H, W) format.
+ """
+ old_h, old_w = original_size
+ new_h, new_w = self.get_preprocess_shape(
+ original_size[0], original_size[1], self.target_length)
+ coords = deepcopy(coords).to(paddle.float)
+ coords[..., 0] = coords[..., 0] * (new_w / old_w)
+ coords[..., 1] = coords[..., 1] * (new_h / old_h)
+ return coords
+
+ def apply_boxes_paddle(self,
+ boxes: paddle.Tensor,
+ original_size: Tuple[int, ...]) -> paddle.Tensor:
+ """
+ Expects a paddle tensor with shape Bx4. Requires the original image
+ size in (H, W) format.
+ """
+ boxes = self.apply_coords_paddle(
+ boxes.reshape([-1, 2, 2]), original_size)
+ return boxes.reshape([-1, 4])
+
+ @staticmethod
+ def get_preprocess_shape(oldh: int, oldw: int,
+ long_side_length: int) -> Tuple[int, int]:
+ """
+ Compute the output size given input size and target long side length.
+ """
+ scale = long_side_length * 1.0 / max(oldh, oldw)
+ newh, neww = oldh * scale, oldw * scale
+ neww = int(neww + 0.5)
+ newh = int(newh + 0.5)
+ return (newh, neww)